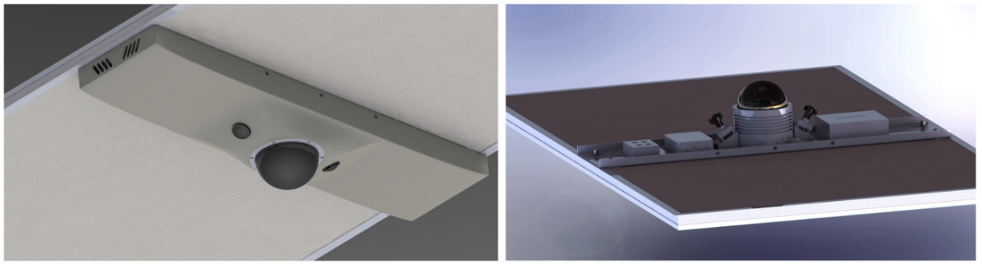
Indoor Infrastructure Sensor Node
Compared with on-board installations, the proposed system will utilize sensor nodes, called ISNs, affixed to the infrastructure (i.e., on the ceiling). This approach has the potential to simplify the design and enhance the system's safety. Each ISN will first calculate the local perception and localization (PL) individually based on the raw data collected from sensors (i.e., Lidar and cameras), where the perception is for the obstacles of all objects in the fleet's driving area and the localization is for the vehicles. This data will then be sent to the cloud for a global PL calculation. Subsequently, this information will be utilized in the decision-making processes and the planning and control module to determine the appropriate control commands for each vehicle, including the steering and speed information. Lastly, these control commands will be transmitted to each indoor vehicle via WIFI. Additionally, the wheel speed and steering angle will be measured using the pre-installed encoders in the motors. These signals will be sent to the cloud via WIFI, serving as supplementary information for the decision-making processes and the planning and control module.
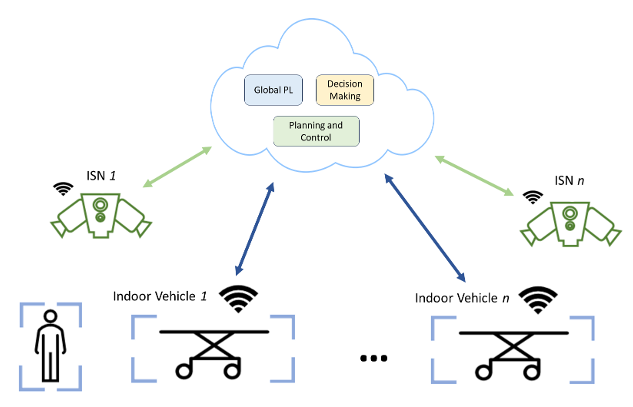
Indoor Framework of 5G Autonomous Fleet Mobility
Indoor Real-Time Perception
Similar to the outdoor perception, indoor perception also consists of Point Cloud Background Subtraction, YOLOv8 Camera Object Detection and Lidar Camera Association. However, the indoor environment is typically more crowded than the outdoor environment, so we improved the camera detection so that it can estimate the object’s 3D position, which will provide another redundancy to the perception system. The YOLOv8 is retrained so that it cannot only detect objects but also the contact points between objects and the ground plane, like the wheels of a chair or the feet of a person. Then the camera intrinsic and extrinsic information are used to estimate the object’s 3D position.
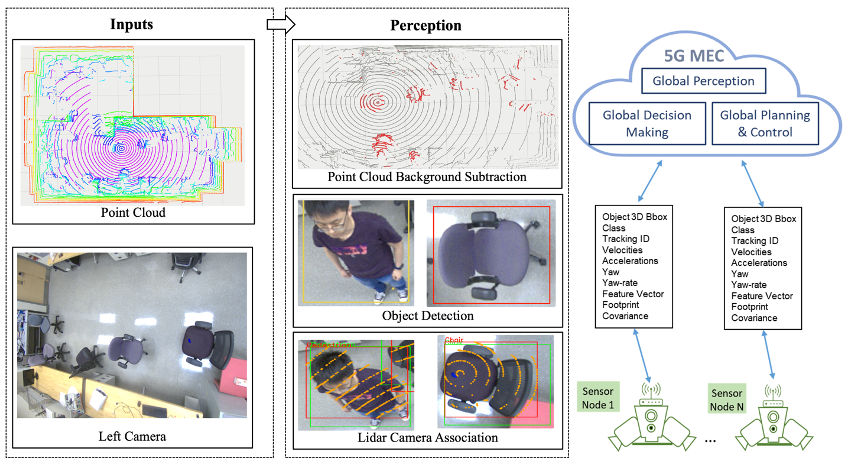
Also, a compact way to represent the safe driving region, named Drivable Space, is proposed. In a crowded environment where obstacles are too close for a robot to pass between them, these occluded regions can be connected and a drivable space where the robot is safe to drive can be extracted.
Experimental studies will be performed using our autonomous hospital beds and carts that have been developed to be utilized with an assistive system or fully autonomous using 5G and cloud computations.
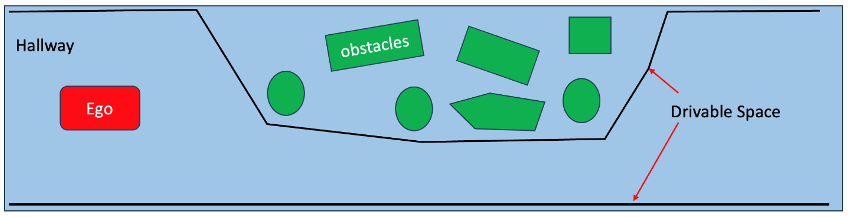