Background
A complex continuous industrial process contains a number of interconnected sub-processes or functional groups which are dynamically inter-related. They usually generate huge amounts of data during daily operations. While countless measurements and data are captured at varying time intervals across the entire process, there is remarkably little information that is used as a diagnostic or system evaluation measure on how well the plant is operating and in what ways the operations could be improved. In the past decade, pattern discovery has been employed in oil sands industries. However, it is essentially designed for static databases. When time-dependent data is involved, the fundamental requirement of pattern associations could not be easily satisfied since stationary characteristics could not be assumed and auto-correlation and cross correlation may change from time to time. These pose a very difficult problem for a dynamic systems.
Description of the invention
This invention demonstrates a new pattern discovery and analysis system for multivariate time series. With this new system, the discovered patterns are used to reveal the local association relations and provide a base to build predictive models for root cause analysis and feed forward control. Experiments on synthetic and real world data show that statistical significant association patterns, known as lead patterns, could be discovered on single sequences or multivariate time series. The locally association patterns can then be synthesized; displayed for system interpretation and modeling and also be used for predictive analysis and more comprehensive online process monitoring and control. It thus greatly reduces the design and operational cost. Given the high cost of unexpected interruption of operations, energy consumption, environmental pollution, and sub-optimal performance in these large facilities, the commercial values of the proposal are enormous.
Advantages
The software platform, known as Intelligent Control and Monitoring (ICM), has been developed to efficiently and effectively handle massive multivariate time series data, enabling automatically to discover temporal pattern associations from multiple TS data, in order to be used for root cause analysis, and to provide prediction module based on the discovered temporal pattern associations. Thus the ICM provides hazard event prevention and automatic monitoring.
Potential applications
- Chemical/industrial automatic process control and optimization
- Electrical meter/smart grid control/analysis
- Financial market and securities analysis
- Analysis of patterns in health care research samples
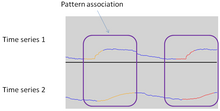
Multivariate Times Series Pattern Discovery
Image: Multivariate Times Series Pattern Discovery
Reference
8810-7253
Inventors
Prof. Andrew K.C. Wong
Dr. Gary Li
Patent status
Issued patents in U.S. and Canada
Stage of development
Prototype built and tested, with ongoing development for multiple applications
Contact
Scott Inwood
Director of Commercialization
Waterloo Commercialization Office
519-888-4567, ext. 43728
sinwood@uwaterloo.ca
uwaterloo.ca/research