Background
Identifying, categorizing and explaining patterns in relational date sets is complex due to a wide range of interrelated and intertwining factors. Thus functional associations are often masked at the data level due to such hidden entanglement. This is a specific problem related to relational data sets in many areas including healthcare, finance and cybersecurity. There is a need to both discover and disentangle patterns inherent in relational data sets in order to surface deeper actionable knowledge in a way that is explainable (i.e. mitigate the AI “black box” confidence issue).
Description of the invention
University of Waterloo (UW) researchers have developed novel PDD (Pattern Discovery and Disentanglement) software to discover deep knowledge inherent in relational and array data for various applications. PDD uses novel autonomous and scalable algorithms to disentangle and discover deep knowledge to reveal subtle functionality and relations and uses the knowledge discovered to enhance machine learning, achieving much better prediction results with explanation. The software also solves imbalanced class, biases, anomaly and outliers problems that have plagued ML for decades.
Advantages
The invention provides:
- Time/Cost Reduction – prediction based only on data with no reliance on explicit prior knowledge.
- Higher accuracy - leveraging rare cases and mislabeling
- Robust to data noise, biases and imbalanced classes
- Flexible - can be applied to a wide range of scenarios
- Explainable –providing explicit patterns/pattern clusters for further exploration, experts’ understanding and knowledge organization.
Potential applications
- Unsupervised classification/tagging of data in relational datasets – as an example, if a tabulated record associated with heart disease (Figure.1) is inputted to PDD software, the output can include:
- Automatic labeling and grouping of patients with explanation
- Identification of correlated indicants for each group
- Identification of Rare Cases and the patterns they possess
- Detection of early stage of the disease in patients
- Prediction of “Healthy” and “Sick” patients and identification of mislabeled, biased cases and outliers.
- Other sectors where relational tables are used, e.g. Finance, Banking, Insurance, Logistics, Manufacturing and Cybersecurity
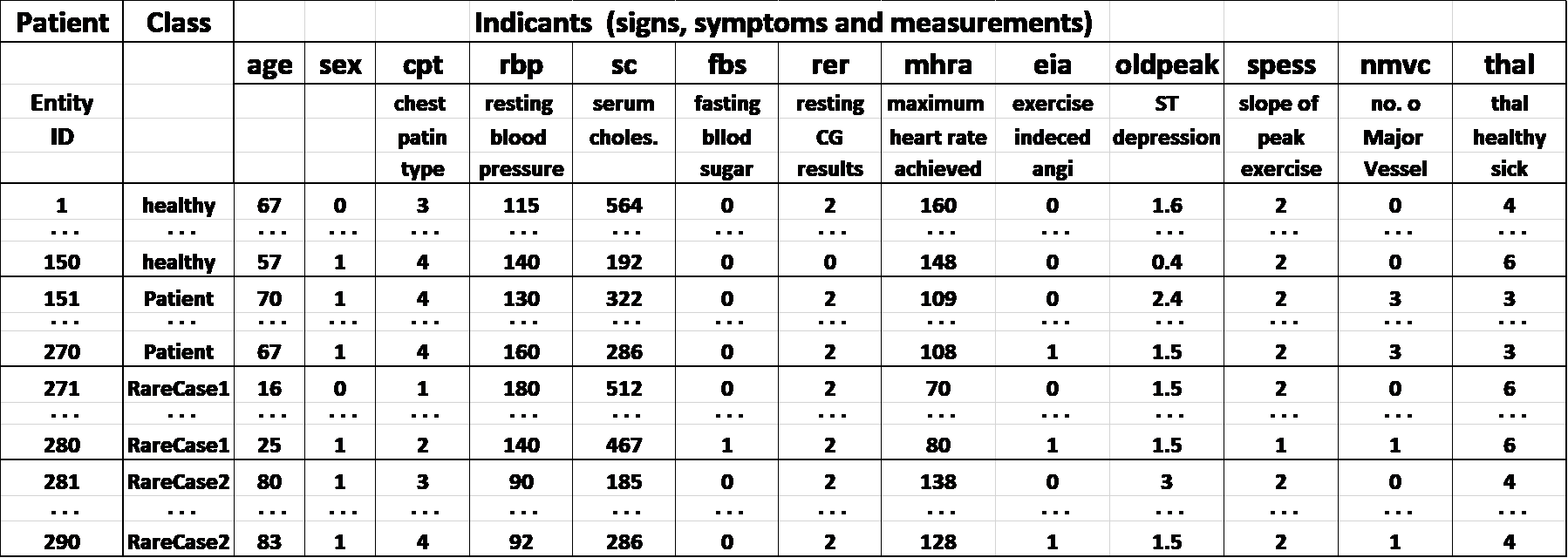
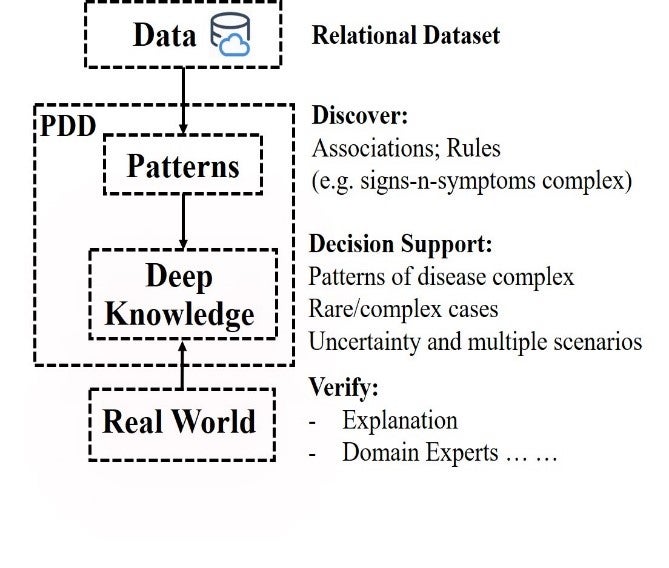
Reference
10146
Patent status
Patent pending
Stage of development
Working server prototype and
validating application data
Contact
Scott Inwood
Director of Commercialization
Waterloo Commercialization Office
519-888-4567, ext. 33728
sinwood@uwaterloo.ca
uwaterloo.ca/research