Welcome to Statistics and Actuarial Science
The Department of Statistics and Actuarial Science is a top-tier academic unit among statistical and actuarial science globally. Our community is engaged in topics such as actuarial science, biostatistics, data science, quantitative finance, statistics, & statistics-computing. Our department is home to more than 60 full-time faculty researching diverse and exciting areas, over 1000 undergraduate students from around the world, and more than 175 graduate students in master, doctoral, and professional programs.
News
Students Tackle Data at 2024 ASA DataFest
From April 26-28, teams of undergraduate student competed at the 2024 American Statistical Association (ASA) DataFest hosted by the Department of Statistics and Actuarial Science. Students worked around the clock with complex datasets assisted by graduate students, faculty and industry professionals to compete for prizes.
Cecilia Cotton and Jordan Hamilton win Distinguished Teacher Awards
Congratulations to Cecilia Cotton and Jordan Hamilton, recipients of the 2024 Distinguished Teacher Awards from Waterloo’s Centre for Teaching Excellence. Since 1975, the award has been given annually to four exemplary instructors from across the university who have a “record of excellent teaching over an extended period at Waterloo, usually at least five years.”
Read the full article on the Faculty of Mathematics website.
Qiuqi Wang Won the 2024 SSC Pierre Robillard Award
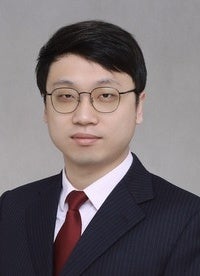
The Statistical Society of Canada (SSC) citation reads: "The Pierre Robillard Award is awarded annually by the SSC to recognize the best Ph.D. thesis in probability or statistics defended at a Canadian university during the previous year.
Qiuqi Wang is the 2024 winner of the Pierre Robillard Award of the Statistical Society of Canada. Qiuqi’s thesis, entitled "Characterizing, optimizing and backtesting metrics of risk", was written while he was a doctoral student at the University of Waterloo working under the supervision of Ruodu Wang."
Read the full article on the SSC website.
Events
Seminar by Babak Shahbaba
Statistics and Biostatistics seminar series
Babak Shahbaba
University of California, Irvine (UCI)
Room: M3 3127