Friday, September 13, 2013 2:30 pm
-
2:30 pm
EDT (GMT -04:00)
Sparsity, boosting and ensemble methods
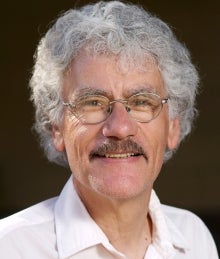
Jerome Friedman, Stanford University
Jerome Friedman is professor of statistics at Stanford University, where he has held a faculty position since 1982. His outstanding contributions to statistical methods and computer science in the areas of nonparametric statistics and machine learning have led to many honours and awards. His two books Classification and Regression Trees (1984, co-authored with Leo Breiman, Richard Olshen, and Charles Stone) and The Elements of Statistical Learning: Data Mining, Inference and Prediction (2001, co-authored with Trevor Hastie and Rob Tibshirani), are among the most widely used in statistics, machine learning, and data mining.
- Everyone welcome.
- Reception will follow in the Bruce White Atrium.
- Co-sponsored by Department of Statistics and Actuarial Science and Google.
See "Sparsity, Boosting and Ensemble Methods" lecture poster (PDF)