Factor Models and PCA in light of the spiked covariance model
Principal components analysis and Factor models are two of the classical workhorses of high-dimensional data analysis, used literally thousands of times a day by data analysts the world over. But now that we have entered the big data era, where there are vastly larger numbers of variables/attributes being measured that ever before, the way these workhorses are deployed needs to change.
In the last 15 years there has been tremendous progress in understanding the eigenanalysis of random matrices in the setting of high-dimensional data in particular progress in understanding the so-called spiked covariance model. This progress has many implications for changing how we should use standard `workhorse' methods in high-dimensional settings. In particular it vindicates Charles Stein's seminal insights from the mid 1950's that shrinkage of eigenvalues of covariance matrices is essentially mandatory, even though today such advice is still frequently ignored. We detail new shrinkage methods that flow from random matrix theory and survey the work of several groups of authors.
About Dr. Donoho:
Da
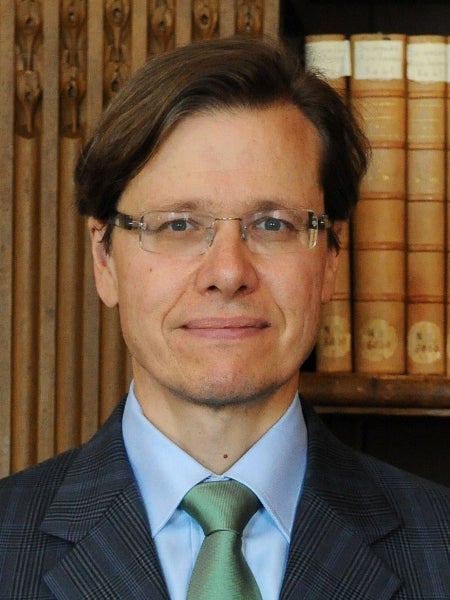
He is a member of the United States National Academy of Sciences as well as a foreign associate of the Academie des Sciences of France and has been named a MacArthur Fellow, a Fellow of the American Academy of Art and Sciences, a Fellow of the Society for Industrial and Applied Mathematics, and a Fellow of the American Mathematical Society. He has received the COPSS Presidents’ Award, the John von Neumann Prize, and the Norbert Wiener Prize. He holds an honorary doctorate from the University of Chicago and in 2013 became a Shaw Prize Laureate in the Mathematical Sciences.
David Sprott Distinguished Lecture by Professor David Donoho Poster (PDF)