Please Note: This seminar will be given in-person.
Distinguished Lecture Claudia Klüppelberg Room: M3 3127 |
Max-linear Graphical Models for Extreme Risk Modelling
Graphical models can represent multivariate distributions in an intuitive way and, hence, facilitate statistical analysis of high-dimensional data. Such models are usually modular so that high-dimensional distributions can be described and handled by careful combination of lower dimensional factors. Furthermore, graphs are natural data structures for algorithmic treatment. Moreover, graphical models can allow for causal interpretation, often provided through a recursive system on a directed acyclic graph (DAG) and the max-linear Bayesian network we introduced in [1] is a specific example. This talk contributes to the recently emerged topic of graphical models for extremes, in particular to max-linear Bayesian networks, which are max-linear graphical models on DAGs.
In this context, the Latent River Problem has emerged as a flagship problem for causal discovery in extreme value statistics. In [2] we provide a simple and efficient algorithm QTree to solve the Latent River Problem. QTree returns a directed graph and achieves almost perfect recovery on the Upper Danube, the existing benchmark dataset, as well as on new data from the Lower Colorado River in Texas. It can handle missing data, and has an automated parameter tuning procedure. In our paper, we also show that, under a max-linear Bayesian network model for extreme values with propagating noise, the QTree algorithm returns asymptotically a.s. the correct tree. Here we use the fact that the non-noisy model has a left-sided atom for every bivariate marginal distribution, when there is a directed edge between the the nodes.
For linear graphical models, algorithms are often based on Markov properties and conditional independence properties. In [3] we characterise conditional independence properties of max-linear Bayesian networks and in my talk I will present some of these results and exemplify the difference to linear networks.
Claudia Klüppelberg
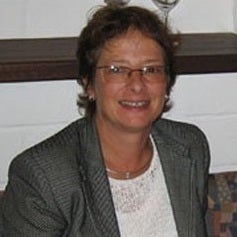
Claudia Klüppelberg received her doctorate from University of Mannheim in 1987 and earned her habilitation in 1993 at the Swiss Federal Institute of Technology in Zurich. In 1997 she became the Chair of the Department of Mathematical Statistics at the Technical University of Munich, and in 2008 she was appointed Carl von Linde Senior Fellow at the Institute for Advanced Study of Technical University of Munich, where she led the Risk Analysis and Stochastic Modeling group. Claudia Klüppelberg is known for her fundamental contributions to extreme value theory and its applications to real life problems. Her research interests combine various disciplines of applied probability theory and statistics with applications in the area of biological, economic and technical risks. In addition to more than 150 publications in scientific journals, Claudia Klüppelberg has coauthored the book Modeling Extremal Events for Insurance and Finance, which currently is in its 10th printing. She is a Medallion Lecturer and an elected Fellow of the Institute of Mathematical Statistics (IMS), a member of the Editorial Board of the Springer Finance book series, and Associate Editor of several scientific journals. She receives an honorary Doctor of Mathematics at the University of Waterloo in 2022.