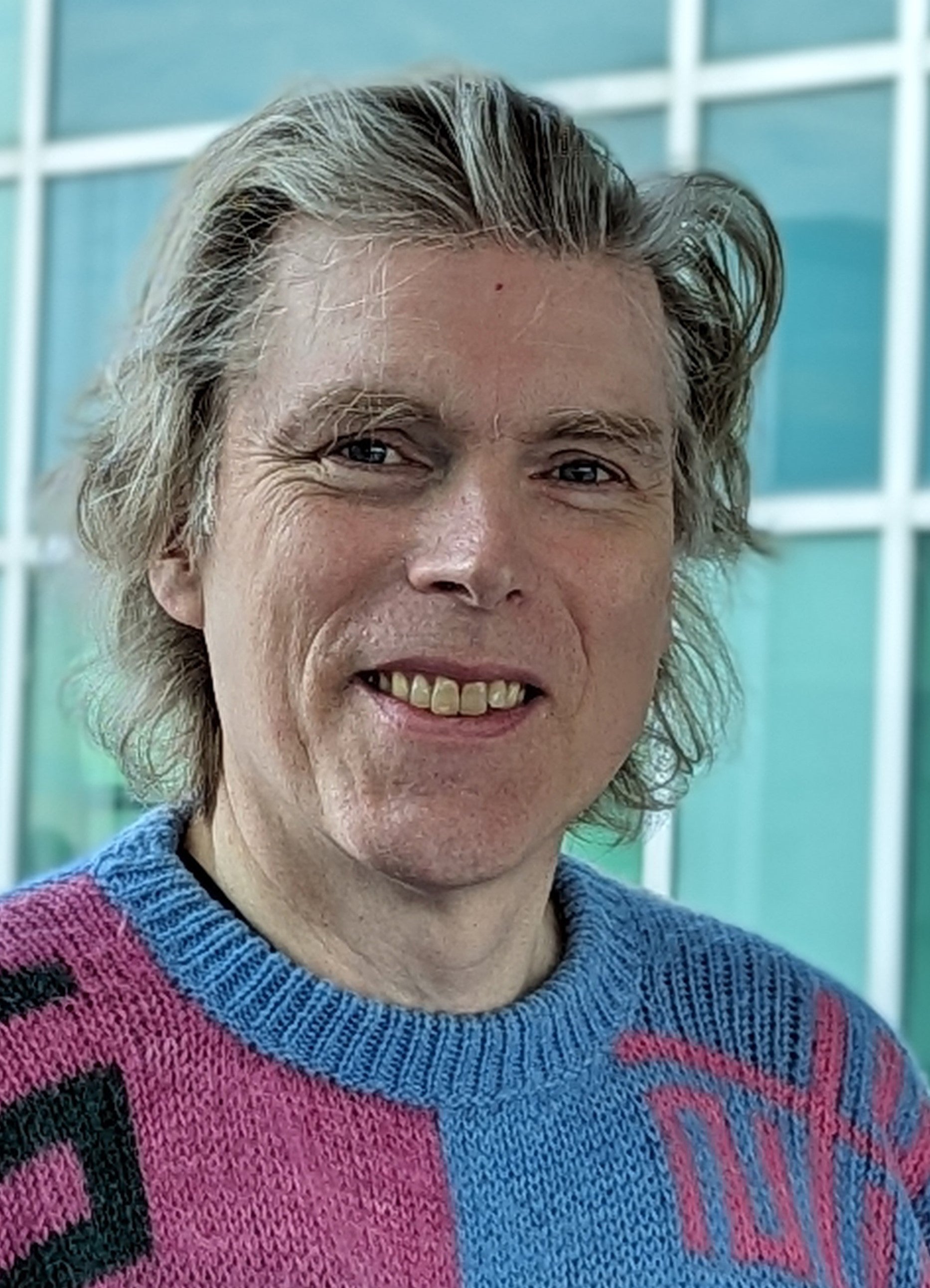
Contact Information:
Matthias Schonlau
Matthias Schonlau's personal website
Research interests
Professor Schonlau's research interests include survey methodology, application of natural language processing to open-ended questions, visualization, and statistical software (Python/ Stata).
Text data from open-ended questions in surveys are frequently ignored in the practice of survey research. Yet open-ended questions are important because they do not constrain respondents’ answer choices. Where open-ended questions are necessary, sometimes multiple human coders hand-code answers into one of several categories. Automated algorithms do not achieve an overall accuracy high enough to entirely replace humans. We classify open-ended questions automatically using text mining for easy-to-classify answers and humans for the remainder. Expected accuracies guide the choice of a threshold delineating between “easy” and “hard”.
This approach has spawned a variety of related projects including: algorithms for automatic occupation coding (categorizing answers to the question “What is your job?” in official surveys); classification of open-ended questions that can take more than one label (equivalent to all-that-apply questions); an algorithm for semi-automatic classification all-that-apply type open-ended questions; training a learning algorithm for double coded data, when such codes are available, and whether or not to purposely double code the training data when there is a fixed budget for human coders.
Visualization: A lot of research has focused on visualizing categorical only data (e.g. bar charts, mosaic plots) and visualizing numerical only data (e.g. scatter plots, histograms, box plots, parallel coordinate plots). But even though most data sets contain mixed categorical/numerical variables, there is much less research that accommodates mixed data types in a single plot. Originally proposed in 2003, Dr. Schonlau’s hammock plot fills that gap. Using visualization of mixed categorical / numerical data as a jumping off point, Dr. Schonlau is currently expanding his research into data visualization.
Education/biography
Professor Schonlau joined the faculty in 2011. From 1999-2011 he was a statistician at RAND corporation and head of the RAND Statistical Consulting Service. He was initially located at RAND's Santa Monica (Los Angeles) headquarters and then moved to RAND's Pittsburgh office. Professor Schonlau spent the academic year 2015/2016 on sabbatical at University of Auckland (New Zealand) and the academic year 2009/2010 on sabbatical at the German Institute for economic analysis (DIW) in Berlin, Germany in cooperation with the Max Planck Institute for Human Development (MPIB). From 1997-1999 Professor Schonlau held a joint appointment with the National Institute of Statistical Sciences and AT&T Labs - Research. He obtained his PhD from the University of Waterloo in 1997 and his master's from Queen's University in 1993. Professor Schonlau grew up in Germany. Professor Schonlau is an elected Fellow of the American Statistical Association and won a 2022 Humboldt Research Prize.
Selected Recent Publications
-
Schonlau, M. Applied Statistical Learning. With Case Studies in Stata, 332 pages. Springer. ISBN 978-3-031-33389-7. (August 2023)
-
Schonlau, M. Hammock plots: visualizing categorical and numerical variables. Journal of Computational and Graphical Statistics, pp 1-16. 2024 (online first)
-
Gweon, H. Schonlau, M. Automated classification for open-ended questions with BERT Journal of Survey Statistics and Methodology. 12(2), 493–504, April 2024.
-
Gweon H., Schonlau M, Wenemark, M. Semi-automated classification for multi-label open-ended questions. Survey Methodology. Dec 2020, 46, 2, 265-282.
-
Schierholz M., Schonlau M. Machine Learning for Occupation Coding - A Comparison Study. Journal of Survey Statistics and Methodology. November 2021, 9(5), pp 1013-1034.
-
Sucholutsky I, Schonlau M. `Less than one'-shot learning: Learning N classes from M < N samples. Proceedings of the thirty-fifth Conference on Artificial Intelligence (AAAI'21). Feb 2021. pp 9739-9746.
-
Sucholutsky I, Schonlau M. Soft-Label Dataset Distillation and Text Dataset Distillation. The International Joint Conference on Neural Networks (IJCNN21). 18-22 July 2021, pp 1-8.