Design team members:
Christopher Jones, David Chow
Supervisor: Professor David Clausi, Professor Philip Howarth
Background
The development of technically advanced tools for use in agriculture is essential to aid farmers in making decisions relating to crop health and resource management. The effective use of hyperspectral imagery in precision farming not only helps farmers to effectively use resources such as herbicides and pesticides, but it also provides insight into the current stage of crop development and health.
Hyperspectral imagery
Generally, hyperspectral images contain radiance or reflectance information in the visible and infrared spectral regions. Figure 1 contains a visual explanation of the composition of a hyperspectral image.
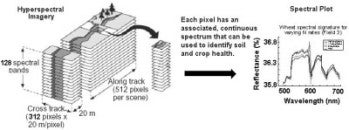
Figure 1: The composition of a hyperspectral image [2].
Precision agriclture
Precision agriculture is the science of improving crop yields and assisting management decisions using high technology sensor and analysis tools. Regardless of the data source, the ultimate goal of precision agriculture remains the same: to assist farmers in the management of their business. Such assistance comes in a variety of forms, but the end result is usually a reduction of required resources. For example, performing a remote sensing analysis of hyperspectral imagery may provide insight into the existence of weeds in a small and localized region of a wheat field. The traditional farmer’s approach to this problem would be to spray the entire field with herbicides ensuring the weed is eradicated from the field. However, with the data from the hyperspectral analysis in hand, the farmer could apply the herbicide to a localized region of the field saving a great deal of time and money.
Nitrogen and water deficiency in wheat
Both nitrogen and water deficiency are serious agricultural problems. An inadequate supply of nitrogen results in chlorophyll deficiency, known as chlorosis, in many crops including wheat. In turn, a lack of chlorophyll reduces the amount of sunlight absorbed by the plants and, thus, results in stunted growth and death. Chlorosis causes the spectral characteristics of the plants to change making it possible for nitrogen deficiency to be detected in hyperspectral imagery. Water deficiency also limits crop development and varies the spectral characteristics of the plants. Figure 2 demonstrates the generalized spectral profile for both healthy and stressed vegetation.
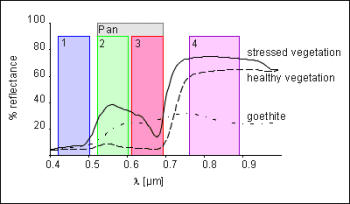
Figure 2: Spectral profile for healthy and stressed vegetation [3].
Project description
Research into the usefulness of hyperspectral imagery for precision agriculture is still in its infancy. While many of the tools developed for use with multispectral images do not currently apply to hyperspectral data, in theory, the multispectral transformations can be extended. In addition, new transformations, specific to hyperspectral data, that exploit the increased information content and higher spectral resolution may provide new insight into agricultural development and should be investigated.
The purpose of this project is twofold. The first objective is to assess the usefulness of hyperspectral imagery in precision agriculture by examining whether two key health indices of wheat crops, nitrogen and water deficiency, can be detected in hyperspectral imagery of a Canadian test farm. The second objective is to explore new hyperspectral image transformations that transform hyperspectral data into a format that maximizes information relevant to precision farming such as crop development and health.
Design methodology
The design methodology to be followed for the project consists of four stages: research, analysis, design, implementation and testing.
Research and setup: the group will develop an in-depth understanding of crop science and remote sensing of hyperspectral data, gaining comprehension of current farming practices in Canada, spectral characteristics of crops and nutrient deficiencies, as well as remote sensing basics, and current remote sensing practices for hyperspectral data. The pre-processing of acquired hyperspectral imagery will also be performed in this stage.
Analysis: the group will apply the knowledge gained during the research phase to a detailed analytical study of hyperspectral imagery. In particular, several pre-processing results, such as the Principal Components Analysis (PCA) and generalized spectral profiles will be studied. By the end of the Analysis phase, the group will have developed a thorough understanding of the application of hyperspectral imagery in the field of precision agriculture.
Design
The group will utilize the knowledge gained from the research and analysis phases to develop a new hyperspectral transformation to maximize information of relevance to precision agriculture from hyperspectral images.
Implementation
The group will implement the hyperspectral transformation according to the specifications laid out in the Design phase. Code optimization will be performed to ensure that the running times of the transformation are as quick as possible. Much of the coding will be done in modules according to the top-down methodology in order to alleviate the need for long and extensive code verification before testing.
Testing
The group will ensure that the hyperspectral transformation created in the implementation phase satisfies the design specifications (and ultimately the workshop’s main objectives). Testing will include the application of the transform to hyperspectral imagery and analysis of results.
Additional resources
Canadian
Center
for
Remote
Sensing
(CCRS)
Education
Section
-
Visit
this
site
for
useful
remote
sensing
tutorials.
Development
of
Remote
Sensing
Image
Products
for
Use
in
Precision
Farming
-
Visit
this
site
for
an
introduction
into
the
potential
uses
of
hyperspectral
imagery
for
precision
farming.
References
[1] Freek D. van der Meer, Imaging Spectroscopy for Geological Applications., 1999.
[2] Oscar Vonder, Center for Geo-Information, Wageningen University, Vegetation – Applications of Present and Future Optical Remote Sensing Satellite Sensors., 1998