Design team members: Dan Holowack and Preethi Vaidyanathan
Supervisors: Dr. Catherine Burns (project advisor)
Background
Most business owners have heard the following recommendation from a social media savvy employee on their staff, ‘We should be on Twitter!’. The business owner answers with a question, ‘Will Twitter drive revenue?’. The true business value of social media continues to be challenged because strategies for clearly measuring performance are fragmented and poorly defined. If only there was a way to directly link social media efforts to product sales. A way to define the dollar value of a single tweet or facebook Page wall post. The Social Media e-Commerce Business Intelligence (SME BI) platform will bridge data between social media engagement, website visitor behaviour and ecommerce store sales to evaluate online initiatives and support business decisions.
Online advertising has significantly impacted the advertising landscape, effectively moving advertising away from print media and onto the World Wide Web. Social Media networking has become a popular tool that businesses are using to reach their customer base. With over 500 million active users on facebook each with an average of 130 friends, and over 190 million visitors per month on Twitter generating in total 65 million Tweets a day, e-Commerce businesses have the potential of reaching a large customer base using Social Media Marketing Techniques. Businesses have always valued Marketing metrics that measure the success of advertising efforts and impacts on sales, however with this new move to Social Media Marketing, much of this information is lost. Currently, there is no effective solution that tells a business how effective their social media efforts are in evaluating how brand awareness is directly impacting business growth.
Project description
This design project will bridge the gap between social media, website analytics and the e-Commerce transactions. The solution will extract key attributes from the business facebook Page and Twitter account to analyze engagement, influence and growth. Combining this information with visitor behaviour on the store website will empower the business to clearly measure how effective their social media marketing methods are and these efforts drive sales.
Design methodology
The system requires four primary data sources, specifically Twitter, facebook, web analytics and the e-Commerce store. Secondary technologies are also necessary to shorten web addresses, track click-through rates and record visitor information. The team is partnered with a local e-Commerce store, All Wet Water Sports (visit all wet water sports' twitter page), to analyze online business sales and track performance of active social media marketing initiatives. A solution will centralize data from several sources as illustrated by the dataflow framework diagram here.
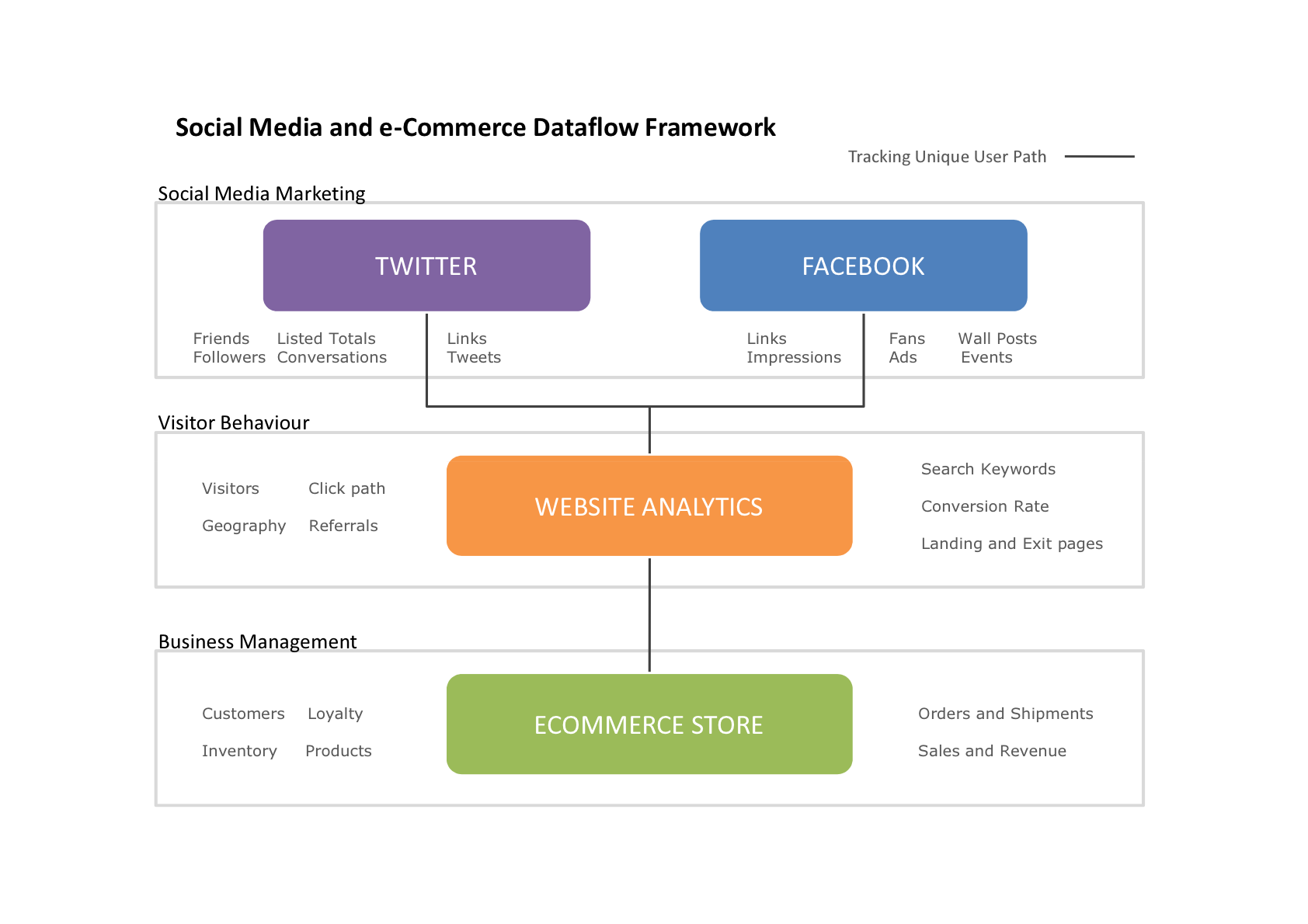
Posts to Twitter and facebook will both contain links leading to internal and external web pages. The internal pages might feature e-Commerce products or sales where external pages share industry news and events. Internal website pages are monitored with web analytics and link tags are used to determine the source of the traffic, from Twitter or Facebook.
Social media information is extracted from the Twitter and Facebook Application Programming Interface (API) at regular intervals. The data storage strategy is subject to API limitations defined by each target service.
A unique attribute is required to join web visitor analytics with store purchases. Visitors are tracked by web analytics and store purchases are recorded by the e-Commerce store. Typically there is no connection between the two. A visitor’s IP Address is the most reliable unique identifier. If an IP Address is logged by the web analytics and at point-of-sale in the e-Commerce store a visitor can be joined to their purchase.
Data from these sources has limited value in raw form. Raw data must be cleansed of irregularities to produce complete and meaningful information. Next this information must be joined as a single relational data model. Establishing these relationships is essential to understand how all four sources will become a one. This final relational data model will be the primary data source for SME BI.
The solution will provide interactive analytics that support advanced segmentation and drill-down functionality. For example, the user may segment metrics by specific products, categories or shipment destination. Drill-down empowers the user to investigate trends from the macro to micro level and identify key factors impacting performance. Historical trending metrics are also necessary to understand purchase patterns and forecast growth. The overall system will enable an e-Commerce storeowner to visualize the relationship between social media marketing and the true ROI of products sold.