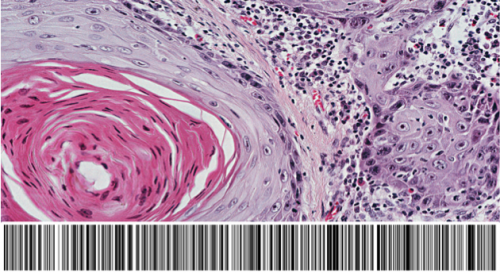
Professor Hamid Tizhoosh has been working on both medical images and artificial neural networks for two decades. Only recently he and his students at the KIMIA Lab were able to exploit the advances in machine learning to tag medical images with ‘deep barcodes’, an idea that can revolutionize diagnostic imaging.
“if we can generate a barcode for each image that uniquely represent that image, let say a mammogram, or a pathology scan, then we can also easily find similar images,” says Tizhoosh. Similar cases retrieved and displayed to clinical experts (e.g., radiologists, pathologists) along with biopsy reports and treatment results can indeed immensely reduce diagnostic error; comparing the image with similar cases from the past is basically “virtual” peer review, a technology that is missing and bitterly needed in medical image analysis.
Tizhoosh was facing some challenges on the way to develop a platform for using deep learning for medical image identification. “Training a deep network with large number of images is a huge problem if you do not have computational resources to do it,” says Tizhoosh. However, he recently received a research award from Microsoft that enables him to use the Microsoft Azure cloud platform for his experiments.
“That’s fantastic news for KIMIA Lab,” he says. “We finally have access to sate-of-the-art GPU and distributed computing to finalize our experiments.” The award enables KIMIA Lab to use the Azure resources equivalent to $20,000 USD.