Waterloo research team partners with Grand River Hospital to perform virtual triaging of spine disorders using AI
Back pain is one of the most common reasons to visit the doctor. In cases of acute pain, disorders, or injury, a healthcare provider will request X-ray images of the spine and pelvis to help make a diagnosis. The images are annotated by hand, where the position and angle of key bony landmarks are measured to indicate if there is a misalignment that requires further treatment or surgery.
A Waterloo research team, led by Dr. John McPhee, professor of systems design engineering (SYDE), is proposing to replace this time-consuming process with artificial intelligence (AI). The team developed a deep learning method of object detection that can locate the required anatomical landmarks, such as the head of the femur bone and points on several vertebrae. The resulting measurements or ‘spinopelvic parameters’ signal the diagnosis in this novel approach to virtual triaging.
The team partnered with Dr. Gemah Moammer, an orthopedic surgeon and head of the spine program at Grand River Hospital (GRH), to compare the accuracy of automatic AI detection with manual measurements. They trained, validated, and tested the deep learning method on 750 lateral spine X-ray images of patients referred to GRH and 250 X-ray images of the lumbar spine and pelvis from Intellijoint Surgical.
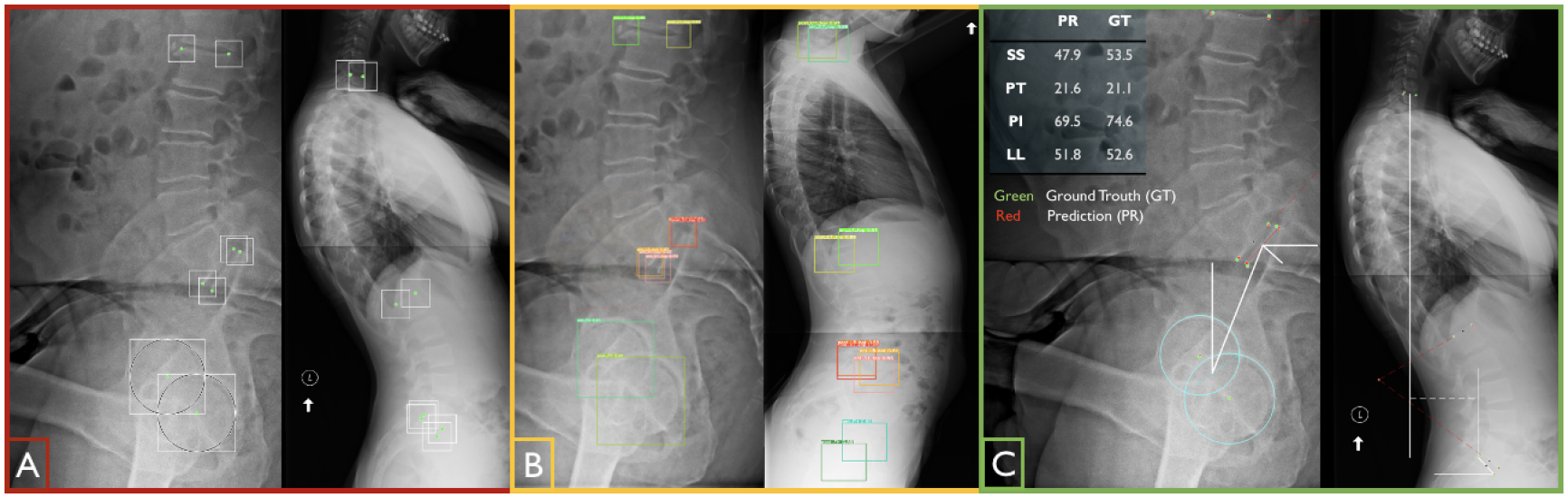
Boundary boxes are placed around landmarks (A) to train the AI model, which can detect the desired landmarks (B) in order to measure spinopelvic parameters (C).
The model’s strong performance is encouraging. The automatically- detected spinopelvic parameters had an average accuracy of 85% compared to manual measurements, which is on par with the agreement between two different radiologists annotating the same X-ray.
The deep learning method also showed promise in overcoming image quality issues that are common in pelvic X-rays. A shield is often used around the pelvis to protect internal organs from radiation but if improperly placed, the shield may cover the required landmarks (e.g. head of femur). The AI model was able to accurately detect landmarks even if obstacles were present in the images.
The results are described in the conference paper Automatic Extraction of Spinopelvic Parameters Using Deep Learning to Detect Landmarks as Objects and were presented by AliAsghar MohammadiNasrabadi, PhD student and research assistant in SYDE, at the Medical Imaging with Deep Learning conference in Zurich, Switzerland in July 2022.