
2023 BIRDSALL-DREISS DISTINGUISHED LECTURE
Old problems, new approach: Applications of Ensemble-Tree Machine Learning to Hydrogeology
Dr. Kenneth Belitz
Research Hydrologist
US Geological Survey
Ensemble tree modeling is a machine learning method well suited for representing complex non-linear phenomena. As such, ensemble tree modeling can be applied to a wide range of questions in hydrogeology, including questions related to hydrogeologic mapping.
Some questions are problems of regression in which one seeks an estimate of a continuous variable. For example, what is the depth to the water table across a region of interest? Other questions are problems of classification. For example, across a region of interest and over a range of depths, is groundwater oxic or reduced?
The U.S. Geological Survey National Water Quality Assessment project (NAWQA) has used ensemble tree methods to address questions related to groundwater quality at regional and national scales. Some of our models evaluate the three-dimensional distribution of factors that can affect groundwater quality, such as pH, redox, and groundwater age. In turn, the modeled factors were used in subsequent models to map the three-dimensional distribution of contaminant concentrations. In our experience, ensemble tree models are a powerful tool for answering difficult questions. They can be used as a complement to process-based modeling and to make predictions at scales that preclude the use of process-based approaches.
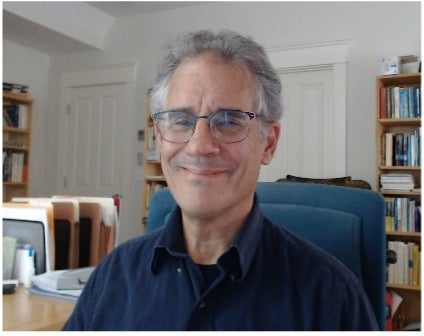
Dr. Kenneth Belitz is a Research Hydrologist in the Water Resources Mission Area of the United States Geological Survey (USGS). He received his B.A. in Geology from Binghamton University, and Ph.D. in hydrogeology from Stanford University in 1985. His dissertation examined the evolution of large-scale groundwater flow in the Denver Basin under the direction of Dr. John Bredehoeft. Throughout his career, Ken has simultaneously pursued two fronts: improving the fundamental hydrogeologic framework of the conterminous U.S., and employing numerical models – and, most recently, machine learning – in novel ways to better understand regional-scale groundwater quality and to project our current understanding into unsampled space.
FREE ADMISSION ~ REFRESHMENTS