Bryan Tripp
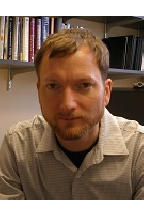
Website
Bryan TrippBiography summary
Professor Tripp uses computational models to study how the brain processes information. He integrates neurobiological models and deep learning to study visuomotor processes. He is also interested in applying these models in challenging robotics tasks, to better understand how the brain deals with the complex physical world. Recent progress in his lab includes: The first deep-network architecture that is based quantitatively on a large cortical network (Tripp, 2019); the most comprehensive model of a higher cortical representation (Rezai et al., 2018); the largest dataset of human-demonstrated robotic grasps (Iyegar et al., 2018); the only robotic head that has movement capabilities on par with humans (including saccade velocity, stereo baseline, and range of motion) (Huber et al., 2018); and the first spiking neural network model of the planning of complex actions (Blouw et al., 2016).
*** I am starting a new research group in Medical AI and have graduate positions open in that area. ***
Research interests
- Computational neuroscience
- Deep learning
- Robotics
- Medical AI
Courses*
- BIOL 487 - Computational Neuroscience
- Taught in 2023
- BME 261 - Prototyping, Simulation and Design
- Taught in 2020, 2021
- BME 355 - Physiological Systems Modelling
- Taught in 2020, 2021
- BME 461 - Biomedical Engineering Design Workshop 2
- Taught in 2023, 2024
- BME 462 - Biomedical Engineering Design Workshop 3
- Taught in 2024, 2025
- SYDE 121 - Digital Computation
- Taught in 2020
- SYDE 552 - Computational Neuroscience
- Taught in 2023
- SYDE 577 - Deep Learning
- Taught in 2024
- SYDE 599 - Special Topics in Systems Design Engineering
- Taught in 2022, 2023
* Only courses taught in the past 5 years are displayed.
Selected/recent publications
- Tripp, BP, Approximating the Architecture of Visual Cortex in a Convolutional Network, Neural Computation, 31(8), 2019, 1551 - 1591
Link - Omid Rezai, Pinar Boyraz Jentsch, Bryan Tripp, A video-driven model of response statistics in the primate middle temporal area, Neural Networks, 108, 2018, 424 - 444
Link - Huber, Scott and Selby, Ben and Tripp, Bryan, OREO: An open-hardware robotic head that supports practical saccades and accommodation,, IEEE Robotics Automation Letters, 3(3), 2018, 2640 - 2645
Link - Selby, Ben and Tripp, Bryan, Extending the Stabilized Supralinear Network model of V1 for binocular image processing, 2017, Neural Networks 90: 29-41.
- Tripp, Bryan and Eliasmith, Chris, Function approximation in inhibitory networks, Neural Networks, 77, 2016, 95 - 106
Link - Nicola, Wilten and Tripp, Bryan and Scott, Matthew, Obtaining Arbitrary Prescribed Mean Field Dynamics for Recurrently Coupled Networks of Type-I Spiking Neurons with Analytically Determined Weights, Frontiers in computational neuroscience, 10, 2016
- Tripp, Bryan P, Surrogate population models for large-scale neural simulations, Neural computation, 2015
Link - Tripp, Bryan, Similarities and differences between stimulus tuning in the inferotemporal visual cortex and convolutional networks, IJCNN, 2017
- Sahand Shaghaghi, Bryan Tripp, Chrystopher Nehaniv, Alexander Mois Aroyo , Kerstin Dautenhahn (2020) FocalVid: Facilitating Remote Studies of Video Saliency. Proc. ACHI 2020, The Thirteenth International Conference on Advances in Computer-Human Interactions, November 21-25 2020 - Valencia, Spain
- S Dutta, B Tripp, GW Taylor, Convolutional Neural Networks Regularized by Correlated Noise, 2018, 15th Conference on Computer and Robot Vision (CRV), 375-382
Link - Peter Blouw
Chris Eliasmith
Bryan Tripp, Proceedings of the 38th Annual Conference of the Cognitive Science Society, 1588 manuscript pages (Accepted in 2016)
Link
Graduate studies
- Currently considering applications from graduate students. A completed online application is required for admission; start the application process now.