Daniel Stashuk
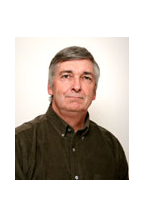
Contact information
Biography summary
Daniel Stashuk is a Professor in the Department of Systems Design Engineering at the University of Waterloo.
His research interests lie in the electrophysiological characterization of neuromuscular disorders, quantitative EMG, EMG signal decomposition, , Decomposition-based Quantitative Electromyography (DQEMG) and EMG signal simulation.
Currently, the major objectives of Professor Stashuk’s ongoing research are to develop and evaluate clinical methods that can assist with the characterization of neuromuscular disorders based on the interpretation of DQEMG results, and to introduce new quantitative statistics that effectively reflect neuromuscular structure and physiology. In addition to his research work, Professor Stashuk has several publications that cover the topics of electromyography and electromyographic signals.
Research interests
- Ergonomics
- Human Factors & Biomedical
- Modeling & Simulation
- Signal & Image Processing
- Decisions Under Uncertainty
- EMG signal simulation
- Machine Intelligence and Knowledge Based Systems
- Adaptive and Nonlinear Systems
- Pattern Analysis
- Quantitative Clinical Diagnosis
- Biosignal detection and analysis Electromyographic (EMG) signal detection and analysis
- Clinical quantitative EMG
- Electrophysiological Characterization of Neuromuscular Disorders
- Clinical Decision Support
- Motor Unit Number Estimation
- Decomposition-based Quantitative Electromyography
Education
- 1985, Doctorate, PhD, McMaster University
- 1982, Master's, MEng, McMaster University
- 1978, Bachelor's, BEng, University of Waterloo
Selected/recent publications
- Raheja, Divisha and Simmons, Zachary and Stashuk, Daniel, Qualitative Review of Quantitative EMG Results Can Improve Needle EDX Studies (P6. 261), Neurology, 86(16 Sup), 2016
- Gilmore, Kevin J and Kimpinski, Kurt and Stashuk, Daniel and Doherty, Tim J and Rice, Charles L, Chronic Inflammatory Demyelinating Polyneuropathy: Muscle Atrophy Associated with Denervation and Neuromuscular Transmission Instability, The FASEB Journal, 30(1 Supp), 2016, 991 - 7
- Power, Geoffrey A and Allen, Matti Douglas and Gilmore, Kevin J and Stashuk, Daniel W and Doherty, Timothy J and Hepple, Russell T and Taivassalo, Tanja and Rice, Charles L, MOTOR UNIT NUMBER AND TRANSMISSION STABILITY IN OCTOGENARIAN WORLD CLASS ATHLETES: CAN AGE-RELATED DEFICITS BE OUTRUN?, Journal of Applied Physiology, 2016
- Piasecki, M and Ireland, A and Stashuk, D and Hamilton-Wright, A and Jones, D and McPhee, J, Age associated motor unit loss is not attenuated by high levels of lifelong exercise, Proceedings of The Physiological Society, 2016
- Ireland, A and Piasecki, M and Coulson, J and Hamilton-Wright, A and Stashuk, D and Jones, D and McPhee, J, Lifelong exercise is associated with greater age-related motor unit remodelling, Proceedings of The Physiological Society, 2016
Graduate studies
- Currently considering applications from graduate students. A completed online application is required for admission; start the application process now.