Researchers at the Institute for Quantum Computing (IQC) have demonstrated a new method, called cycle benchmarking, to assess scalability and compare capabilities of different quantum computer platforms.The finding leads the way towards establishing standards for quantum computing performance and strengthens the global effort to build a large-scale, practical quantum computer.
“A consistent method for characterizing and correcting the errors in quantum systems provides standardization for the way a quantum processor is assessed, allowing progress in different architectures to be fairly compared,” said Joel Wallman, Assistant Professor at IQC and the Department of Applied Mathematics at the University of Waterloo.
Cycle benchmarking provides a solution that helps quantum computing users determine the comparative value and increase the capability of any hardware platform to deliver robust solutions for their applications of interest. The breakthrough comes as the quantum computing race is rapidly heating up and the number of cloud quantum computing platforms and offerings is rapidly expanding. In the past month alone there have been major announcements from Microsoft, IBM and Google.
This method also determines the probability of an error under various quantum computing applications, when the application is implemented through randomized compiling. This means that cycle benchmarking provides a cross-platform means of measuring and comparing the capabilities of quantum processors offered from different providers, in a way that can be customized to users’ applications of interest.
Cycle benchmarking unlocks the door to assessing, improving and validating quantum computing capabilities in the current era of quantum discovery, where error-prone quantum computers hope to deliver new solutions to pressing problems and the quality of these solutions that can no longer be verified by high-performance computers, says Joseph Emerson, faculty member at IQC and the applied mathematics department and co-author of the study.
Emerson and Wallman founded the IQC spin-off Quantum Benchmark Inc., which has already licensed this technology to several world-leading quantum computing providers, including Googles Quantum AI effort.
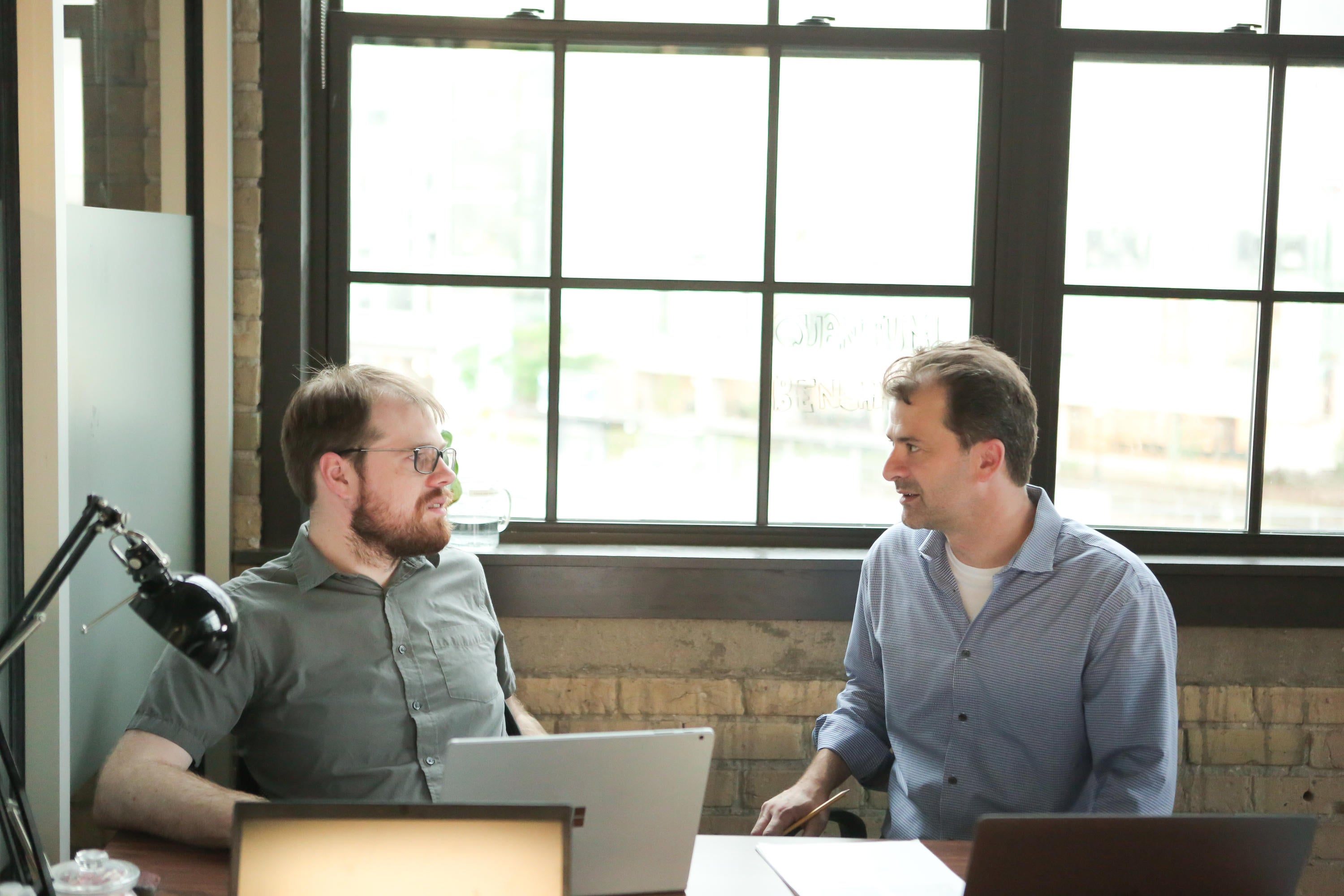
IQC faculty members Joel Wallman and Joseph Emerson at the offices of Quantum Benchmark.
The error problem
Quantum computers offer a fundamentally more powerful way of computing, thanks to quantum mechanics. Compared to a traditional or digital computer, quantum computers can solve certain types of problems more efficiently. However, qubits—the basic processing unit in a quantum computer—are fragile; any imperfection or source of noise in the system can cause errors that lead to incorrect solutions under a quantum computation.
Gaining control over a small-scale quantum computer with just one or two qubits is the first step in a larger, more ambitious endeavour. A larger quantum computer may be able to perform increasingly complex tasks, like machine learning or simulating complex systems to discover new pharmaceutical drugs. Engineering a larger quantum computer is challenging; the spectrum of error pathways becomes more complicated as qubits are added and the quantum system scales.
To scale or not to scale
Characterizing a quantum system produces a profile of the noise and errors, indicating if the processor is actually performing the tasks or calculations it is being asked to do. To understand the performance of any existing quantum computer for a complex problem or to scale up a quantum computer by reducing errors, it’s first necessary to characterize all significant errors affecting the system.
Wallman, Emerson and a group of researchers at the University of Innsbruck identified a method to assess all error rates affecting a quantum computer. They implemented this new technique for the ion trap quantum computer at the University of Innsbruck, and found that error rates don’t increase as the size of that quantum computer scales up, a very promising result.
“Cycle benchmarking is the first method for reliably checking if you are on the right track for scaling up the overall design of your quantum computer,” said Wallman. “These results are significant because they provide a comprehensive way of characterizing errors across all quantum computing platforms.”
The paper Characterizing large-scale quantum computers via cycle benchmarking was published in Nature Communications on November 25.