A light emitting diode (LED)-based spectrophotometer designed and implemented by Institute for Quantum Computing (IQC) researchers is the first demonstration of characterizing optical coatings using a simple, automated device.
Optical coatings are thin layers of film that manufacturers put on optical components such as mirrors, glasses, bank notes and camera lenses to alter the way that particular wavelengths of light are transmitted or reflected. Characterizing the coatings describes what film is adhered to the optical component and provides the right information so the user knows what affect the optical component will have on the light.
The peer-reviewed paper Inexpensive LED-Based Optical Coating Sensor, published in the October 1 issue of IEEE Sensors, is the first for Kayla Hardie, who started the project as an undergraduate research assistant during her first year of studies at the University of Waterloo. In search of a meaningful work experience that would enhance her academic career, Hardie approached IQC faculty member Thomas Jennewein about joining his research group.
“Professor Jennewein was open-minded about what I could do, despite the fact that I was a first-year student,” said Hardie. “Everyday I faced a new learning curve in the lab. I was part of the full cycle – from the initial idea, to building the device, and writing and submitting the paper.”
Optics labs often contain a collection of miscellaneous mirrors and lenses with different types of coatings, however many are either missing labels or have potentially degraded over time. The optics lab that Hardie was working in was no exception. She was challenged to build a useful laboratory tool to sort and identify the collection of optical lenses. She created the LED-based spectrophotometer device that characterizes the transmission spectra of optical components in an efficient and reliable manner.
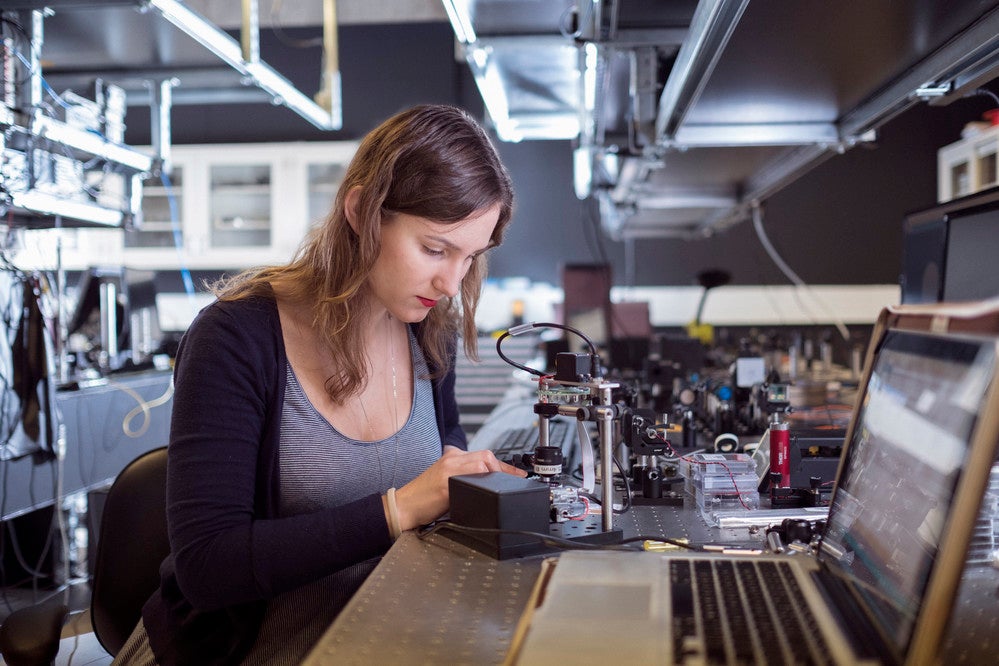
Kayla Hardie working with the LED-based spectrophotometer in the lab.
Run by an Arduino Uno microcontroller, the spectrophotometer – also called an optical coating sensor – rotates 10 LEDs over the optical test sample. A silicon photodetector measures the light transmitted through the sample, identifying the optical coating of the sample based on the amount of transmitted light. The 10 LEDs cover a spectrum from ultraviolet (365 nm) to near-infrared (1,000 nm), providing a wide range of wavelengths that cover the most commonly used coating types found in optics laboratories. Advantages of using LEDs include their compact size, low requirements for warm up time and power, and affordability.
Hardie and her collaborators, including Jennewein, postdoctoral fellow Katanya Kuntz and PhD student Sascha Agne, developed a user interface to control the device to perform calibration measurements in addition to characterizing the optical coating of a sample. “It can be very difficult to find optical filters that only transmit the desired wavelength range and completely block unwanted spectra,” explained Hardie. “We used a simple calibration method to completely eliminate any unwanted wavelengths from getting through, an option not possible with current commercial optical filters.”
The optical coating sensor could make an excellent teaching tool in the classroom and is an affordable alternative to commercial-grade spectrophotometers, essential for use in the lab. “This device is automated, portable, inexpensive, user-friendly and simple to build,” said Hardie. Increasing the number of LEDs, as well as choosing LEDs with smaller emission angles to enhance the robustness of the sensor during alignment are possible future improvements. So far, the optical coating sensor has demonstrated its potential to become a valued tool in any optics lab.