Chris Eliasmith, PEng
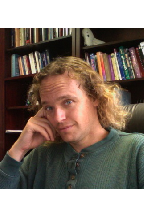
Professor, Canada Research Chair
Email: celiasmi@uwaterloo.ca
Location: E7 6324
Phone: 519-888-4567 x42638
Biography
Chris Eliasmith is a Professor jointly-appointed in the Systems Design Engineering department and the Philosophy department, and is also cross-appointed to Computer Science at the University of Waterloo. He is the Founding Director of the Centre for Theoretical Neuroscience at Waterloo, as well as the Canada Research Chair in Theoretical Neuroscience. The Centre focuses on mathematical characterizations of a variety neural systems, from individual ion channels to large-scale networks.
Professor Eliasmith heads the Computational Neuroscience Research Group (CNRG), which is developing a framework for modelling the function of complex neural systems (the Neural Engineering Framework or NEF). The NEF is grounded in the principles of signal processing, control theory, statistical inference, and good engineering design, while providing a rational and robust strategy for simulating and evaluating a variety of biological neural circuit functions. Members of the group apply the NEF to projects characterizing sensory processing, motor control, and cognitive function.
Professor Eliasmith and his team have also proposed the Semantic Pointer Architecture (SPA) for building large-scale models of biological cognition. Using those methods his team successfully built the world’s largest functional simulation of the human brain – Semantic Pointer Architecture Unified Network (Spaun). Spaun consists of 6 million simulated neurons and over 20 billion connections, enabling it to perform 12 tasks such as copy drawing, counting, instruction following, answering questions and fluid reasoning. It has a digital eye that is used for visual input and a simulated muscle-controlled arm used for drawing its responses.
Work at the CNRG is divided into applications and theoretical development. Theoretical work includes extending the NEF to be more general (e.g. account for a wider range of single cell dynamics), more biologically plausible (e.g., capture network physiology and topology more precisely), and more adaptive (e.g., including better adaptive filtering, learning, etc.). This theoretical work has lead to innovations including the Legendre Memory Unit (LMU) and Spatial Semantic Pointers (SSPs), both of which have been used to improve on a wide variety of state-of-the-art results in machine learning. CNRG members are exploring neural functions to explain not only how neural systems implement complex dynamics (the focus of the NEF), but also what neural systems are designed to do in general – i.e., what the basic functional principles of the brain are.
On the more applied side, the CNRG is building complex single cell networks to test hypotheses about the functioning of a given neural system as well as artificial intelligence and robotics applications. In the case of neural simluations, simulation results are compared against available neural and behavioural data, and are then used to make novel predictions. In the case of pure machine learning or robotics problems, the focus is on setting new state-of-the-art results or providing new capabilities. CNRG members have constructed models of nonlinear adaptive arm control, working memory, locomotion, decision making, quadcopter control, the basal ganglia (implicated in Parkinsons Disease), rodent navigation, large language models, and language use. Lab members regularly use neuromorphic hardware to demonstrate the advantages of such approaches in practice.
Professor Eliasmith is the author of “How to build a brain: A neural architecture for biological cognition” and co-author of “Neural Engineering: Computation, representation and dynamics in neurobiological systems”.
Professor Eliasmith heads the Computational Neuroscience Research Group (CNRG), which is developing a framework for modelling the function of complex neural systems (the Neural Engineering Framework or NEF). The NEF is grounded in the principles of signal processing, control theory, statistical inference, and good engineering design, while providing a rational and robust strategy for simulating and evaluating a variety of biological neural circuit functions. Members of the group apply the NEF to projects characterizing sensory processing, motor control, and cognitive function.
Professor Eliasmith and his team have also proposed the Semantic Pointer Architecture (SPA) for building large-scale models of biological cognition. Using those methods his team successfully built the world’s largest functional simulation of the human brain – Semantic Pointer Architecture Unified Network (Spaun). Spaun consists of 6 million simulated neurons and over 20 billion connections, enabling it to perform 12 tasks such as copy drawing, counting, instruction following, answering questions and fluid reasoning. It has a digital eye that is used for visual input and a simulated muscle-controlled arm used for drawing its responses.
Work at the CNRG is divided into applications and theoretical development. Theoretical work includes extending the NEF to be more general (e.g. account for a wider range of single cell dynamics), more biologically plausible (e.g., capture network physiology and topology more precisely), and more adaptive (e.g., including better adaptive filtering, learning, etc.). This theoretical work has lead to innovations including the Legendre Memory Unit (LMU) and Spatial Semantic Pointers (SSPs), both of which have been used to improve on a wide variety of state-of-the-art results in machine learning. CNRG members are exploring neural functions to explain not only how neural systems implement complex dynamics (the focus of the NEF), but also what neural systems are designed to do in general – i.e., what the basic functional principles of the brain are.
On the more applied side, the CNRG is building complex single cell networks to test hypotheses about the functioning of a given neural system as well as artificial intelligence and robotics applications. In the case of neural simluations, simulation results are compared against available neural and behavioural data, and are then used to make novel predictions. In the case of pure machine learning or robotics problems, the focus is on setting new state-of-the-art results or providing new capabilities. CNRG members have constructed models of nonlinear adaptive arm control, working memory, locomotion, decision making, quadcopter control, the basal ganglia (implicated in Parkinsons Disease), rodent navigation, large language models, and language use. Lab members regularly use neuromorphic hardware to demonstrate the advantages of such approaches in practice.
Professor Eliasmith is the author of “How to build a brain: A neural architecture for biological cognition” and co-author of “Neural Engineering: Computation, representation and dynamics in neurobiological systems”.
Research Interests
- Theoretical Neuroscience, Computational Neuroscience, Neuromorphics, Spiking Neural Networks, Cognitive Modeling, Human Factors & Biomedical, Modeling & Simulation, Pattern Analysis, Machine Intelligence & Robotics, Signal & Image Processing, Operational Artificial Intelligence, Robotics
Education
- 2000, Doctorate Philosophy-Neuroscience-Psychology Program, Washington University in St. Louis, USA
- 1995, Master's M.A. Philosophy, University of Waterloo, Canada
- 1994, Bachelor's B.A.Sc. Systems Design Engineering, First Class Honours, University of Waterloo, Canada
Awards
- 2015 NSERC Polanyi Prize - A single national award given annually in recognition of a recent outstanding advance in any NSERC-supported field.
Teaching*
- COGSCI 300 - Intelligence in Machines, Humans, and Other Animals
- Taught in 2023
- PHIL 256 - Introduction to Cognitive Science
- Taught in 2020
- PHIL 356 - Intelligence in Machines, Humans, and Other Animals
- Taught in 2023
- PSYCH 256 - Introduction to Cognitive Science
- Taught in 2020
- SYDE 556 - Simulating Neurobiological Systems
- Taught in 2019, 2022, 2023
- SYDE 750 - Topics in Systems Modelling
- Taught in 2022, 2023
* Only courses taught in the past 5 years are displayed.
Selected/Recent Publications
- Voelker, Aaron R and Eliasmith, Chris, Improving spiking dynamical networks: Accurate delays, higher-order synapses, and time cells, Neural computation, 569, 2018
- Blouw, Peter and Eliasmith, Chris, Using Neural Networks to Generate Inferential Roles for Natural Language, Frontiers in psychology, 2335, 2017
- Kajić, Ivana and Gosmann, Jan and Stewart, Terrence C and Wennekers, Thomas and Eliasmith, Chris, A spiking neuron model of word associations for the Remote Associates Test, Frontiers in psychology, 99, 2017
- Rasmussen, Daniel and Voelker, Aaron and Eliasmith, Chris, A neural model of hierarchical reinforcement learning, PloS one, , 2017
- Crawford, Eric and Gingerich, Matthew and Eliasmith, Chris, Biologically Plausible, Human-Scale Knowledge Representation, Cognitive science, 782, 2016
- Hunsberger, Eric and Eliasmith, Chris, Training spiking deep networks for neuromorphic hardware, arXiv preprint arXiv:1611.05141, , 2016
- Bekolay, Trevor and Bergstra, James and Hunsberger, Eric and DeWolf, Travis and Stewart, Terrence C and Rasmussen, Daniel and Choo, Xuan and Voelker, Aaron and Eliasmith, Chris, Nengo: a Python tool for building large-scale functional brain models, Frontiers in neuroinformatics, 48, 2014
- Tripp, B. and C. Eliasmith, Neural populations can induce reliable postsynaptic currents without observable spike rate changes or precise spike timing., Cerebral Cortex, 1830, 2007
- Parisien, C., C. H. Anderson, and C. Eliasmith, Solving the problem of negative synaptic weights in cortical models., Neural Computation, 0, 0000
- Singh, R. and C. Eliasmith, Higher-dimensional neurons explain the tuning and dynamics of working memory cells, Journal of Neuroscience, 3667, 2006
In The News
Graduate studies
- Currently considering applications from graduate students. A completed online application is required for admission; start the application process now.
- Has Approved Doctoral Dissertation Supervisor (ADDS) status