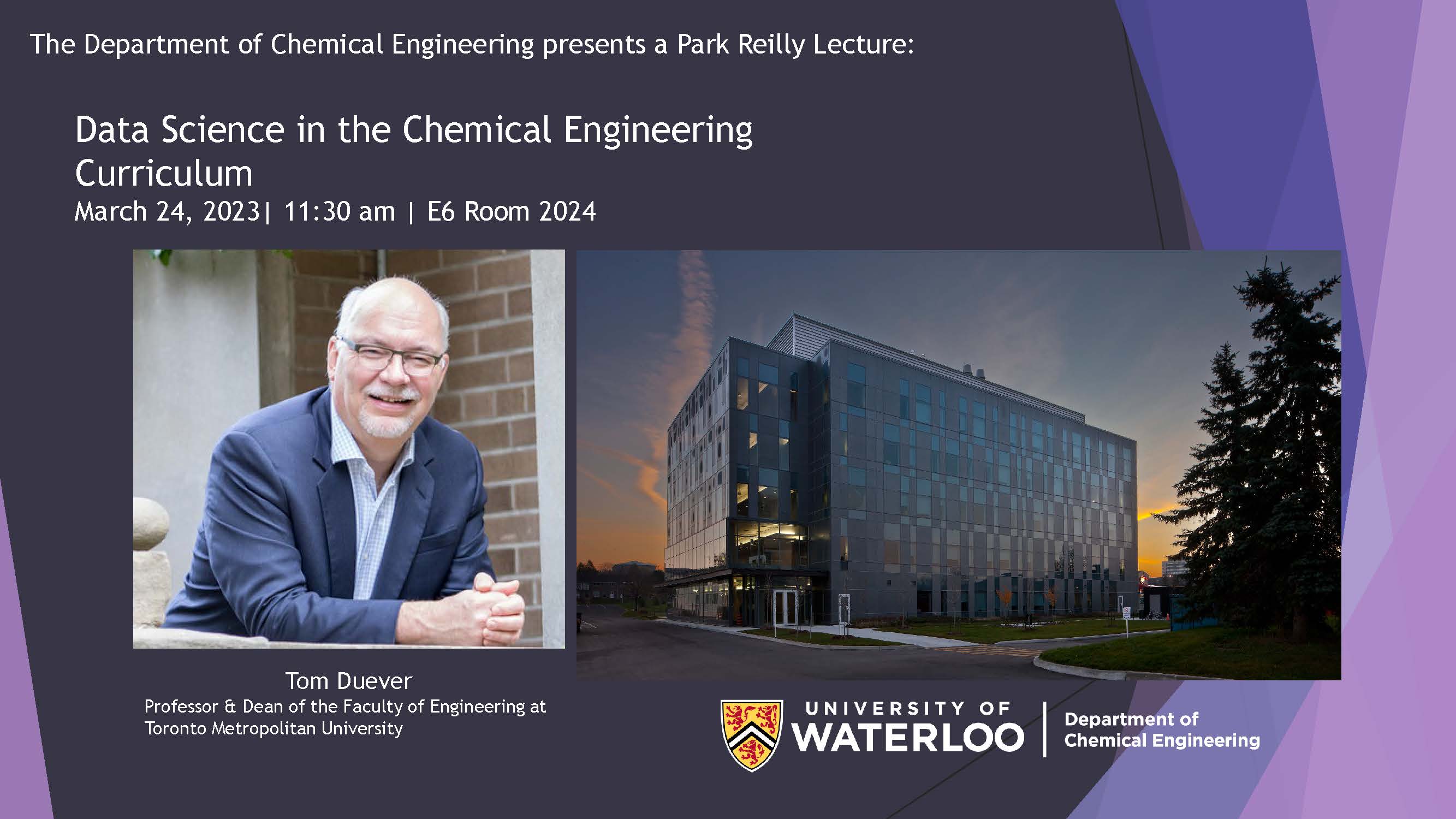
Abstract:
Data Science in the Chemical Engineering Curriculum
The
terms
“Data
Science”,
“Big
Data”
and
“Data
Analytics”
are
becoming
pervasive
affecting
many
aspects
of
our
lives,
many
professional
disciplines
and
certainly
the
profession
of
chemical
engineering.
The
reason
for
this
is
of
course
the
increasing
availability
of
data
brought
on
by
the
proliferation
of
inexpensive
sensors
and
instrumentation,
new
measurement
capabilities
related
to
the
developments
of
the
Internet
of
Things
and
smart
sensors,
and
improved
data
storage
power
like
cloud
computing.
Large
amounts
of
data
are
therefore
readily
available.
Broadly
speaking
Data
Science
can
be
divided
into
three
components,
namely
data
management,
statistical
and
machine
learning,
and
visualization.
It
is
an
interdisciplinary
field
that
uses
scientific
methods,
processes,
algorithms,
and
systems
to
extract
knowledge
and
draw
conclusions
from
data.
Some
view
the
term
data
science
to
be
synonymous
with
statistics.
Others
view
this
as
a
burgeoning
new
discipline
and
compare
the
emergence
of
data
science
with
the
dawn
of
computer
science.
There
are
of
course
many
examples
of
the
use
of
data
science
methodologies
in
the
chemical
engineering
community
including
multivariate
analysis,
on-line
fault
detection,
inferential
sensors,
batch
data
analytics,
experimental
design
approaches,
parameter
estimation
and
model
discrimination.
Applications
range
from
materials
manufacturing
to
pharmaceutical
production,
oil
and
gas,
pulp
and
paper
and
many
others.
Historically,
chemical
engineering
undergraduate
programs,
certainly
in
Canada,
have
incorporated
some
form
of
statistical
training.
This
has
been
accomplished
by
incorporating
one
or
more
courses
in
applied
statistics
into
the
undergraduate
core
curriculum,
in
many
cases
taught
by
chemical
engineering
faculty.
However,
it
can
be
argued
that
chemical
engineering
education
and
training
may
not
have
kept
pace
with
the
proliferation
of
data
described
above
and
that
therefore
techniques
from
a
data-poor
era
are
being
used
to
extract
information
from
data
available
today
from
plants,
refineries
and
data-rich
experiments.
The
challenge
then
is
what
can
be
done
to
better
prepare
graduate
chemical
engineers
for
the
realities
of
today
when
it
comes
to
data
analysis?
Biography:
Tom
Duever
is
Dean
of
the
Faculty
of
Engineering
and
Architectural
Science
and
a
Professor
of
Chemical
Engineering
at
Toronto
Metropolitan
University.
Prior
to
his
role
at
TMU,
Dr.
Duever
served
as
chair
of
the
University
of
Waterloo’s
Department
of
Chemical
Engineering
for
nine
years,
navigating
the
department
toward
unprecedented
growth.
He
has
also
taught
industrial
short
courses
in
experimental
design
and
polymer
reaction
engineering.
Dr.
Duever
is
an
accomplished
researcher
with
interests
including
applied
statistics,
experimental
design,
polymer
reaction
engineering
and
product
development.
He
has
more
than
100
articles
in
journals
and
conference
proceedings
to
his
credit
and
has
supervised
the
research
projects
of
over
40
graduate
students.
Dr.
Duever
is
a
registered
Professional
Engineer
in
the
Province
of
Ontario,
a
Fellow
of
the
Chemical
Institute
of
Canada
and
a
Fellow
of
the
Canadian
Academy
of
Engineering.
He
holds
Bachelor,
Master’s
and
PhD
degrees
in
chemical
engineering
from
the
University
of
Waterloo,
where
he
completed
his
graduate
work
under
the
supervision
of
the
late
Professor
Park
M.
Reilly.