Researcher aims to combat wildfires with Chemical Engineering
This summer Southern Ontario residents experienced the decreasingly rare occurrence of smoke from wildfires adversely affecting the air quality. The climate emergency is ramping up and wildfires are an inevitable outcome. This year’s wildfire season started early and has already burned an unprecedented area of Canadian forests, destroying homes and businesses in its unrelenting path.
Wildfires are difficult to predict. Firefighters often use treatments such as prescribed burns or controlled burns in which firefighters preemptively burn a small piece of forest to manage wildfire risk and attempt to reduce the hazards associated with fires. Firefighters require accurate predictions of a fire’s trajectories, weather patterns, smoke composition and many other factors to make crucial decisions in preventing and combating wildfires.
A new Chemical Engineering faculty member, Professor Joshua Pulsipher is applying his expertise in Computational Chemical Engineering to identify more effective ways to prevent and mitigate wildfires. Pulsipher uses state-of-the-art simulation models to predict and advanced optimization methods to control wildfire risk. He utilizes the chemical engineering concept of transport phenomena in his analysis. This is important, as within wildfires there are chemical reactions and chemical diffusion together.
Two different models are critical for predicting wildfire behaviour. The first is the wildfire combustion model, which typically relies on complex multi-scale reaction and diffusion phenomena that are highly random and rely on several other factors such as foliage conditions. There is also a climate and weather model which describes local weather patterns such as wind and humidity that significantly influence wildfires. These models are highly interconnected as wildfires and weather affect each other. However, the current practice is to use these separately because they both are highly resource and time-intensive to run.
Professor Joshua Pulsipher
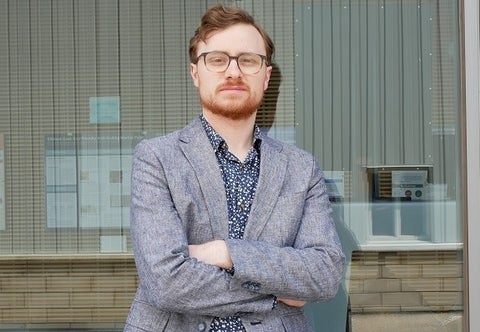
Pulsipher’s approach is novel as he will use state-of-the-art machine learning models to create scalable surrogate wildfire and weather models that he can embed simultaneously into decision-making tools to identify better-informed and more effective fire-management strategies.
Pulsipher aims to develop physics-informed neural operator models specifically designed for complicated space-time models that can rapidly predict wildfire behaviour. The integration of weather behaviour enables these models to also consider factors such as the impact of smoke from controlled burns on populated areas, as well as other prevention and mitigation policies.
“Our modelling approach will look at population centers, the impact smog has on their health, we can consider all these different factors so that we can have a better, wider, more complete picture of what's going on and make more informed decisions,” says Pulsipher.
Pulsipher began this research at Carnegie Mellon University working with Professors Coty Jen and Carl Laird. Jen examines how particles from smoke impact weather and climate. Pulsipher is in the initial stages of this promising research which strives to design the next generation of wildfire decision-making tools that leverage data-driven models to proactively and sustainably mitigate the destruction caused by wildfires.