John Quilty, PhD, PEng
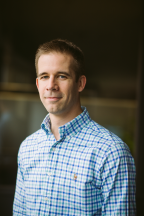
Assistant Professor, Sinnathamby Professor in AI for Sustainable Solutions
Email: jquilty@uwaterloo.ca
Biography
John Quilty is an Assistant Professor in the Department of Civil and Environmental Engineering at the University of Waterloo and the Sinnathamby Professor in AI for Sustainable Solutions.
His research seeks to address the issues of nonlinearity, multiscale change, and uncertainty in hydrological and water resources forecasting. In this regard, Prof. Quilty has introduced new and computationally efficient information-theoretic methods for nonlinear input variable selection considering uncertainty, developed a set of best practices for wavelet-based forecasting of multiscale processes, and proposed a multiscale stochastic data-driven forecasting framework that accounts for uncertainty in each stage of the forecast design, a particularly useful method for creating ensemble forecast systems that can simultaneously perform ensemble member selection and weighting.
He is currently pursuing three research areas that explore: i) new AI, machine learning, and deep learning approaches with a focus on computational efficiency; ii) emerging uncertainty estimation methods (e.g., for input data, input variable selection, parameter, model structure, model output); and iii) coupling physical, conceptual, and data-driven models in addition to auxiliary input sources such as Numerical Weather Predictions, large scale climate indicators, remote sensing, etc. to improve hydrological and water resources forecast accuracy and reliability.
An underlying goal of Prof. Quilty’s research program is to create a unified data-driven framework drawing on Big Data to optimize, plan, and manage urban water resources systems (water supply, wastewater, and stormwater systems), and automatically adapt to environmental and policy changes in the face of uncertainty.
Prior to joining the Department of Civil and Environmental Engineering, Prof. Quilty worked as a Research Associate at McGill University where he focused on new AI-based methods for hydrological forecasting. At the same time, he worked as a Water Meter Operations Engineer at the City of Ottawa where he focused on: project/program management, developing water consumption forecasting models for the purpose of establishing water, wastewater, and storm water rates, analysis of water consumption profiles for design, operational, and billing concerns, water meter right sizing, and the development of specifications and standards for water supply system components.
His research seeks to address the issues of nonlinearity, multiscale change, and uncertainty in hydrological and water resources forecasting. In this regard, Prof. Quilty has introduced new and computationally efficient information-theoretic methods for nonlinear input variable selection considering uncertainty, developed a set of best practices for wavelet-based forecasting of multiscale processes, and proposed a multiscale stochastic data-driven forecasting framework that accounts for uncertainty in each stage of the forecast design, a particularly useful method for creating ensemble forecast systems that can simultaneously perform ensemble member selection and weighting.
He is currently pursuing three research areas that explore: i) new AI, machine learning, and deep learning approaches with a focus on computational efficiency; ii) emerging uncertainty estimation methods (e.g., for input data, input variable selection, parameter, model structure, model output); and iii) coupling physical, conceptual, and data-driven models in addition to auxiliary input sources such as Numerical Weather Predictions, large scale climate indicators, remote sensing, etc. to improve hydrological and water resources forecast accuracy and reliability.
An underlying goal of Prof. Quilty’s research program is to create a unified data-driven framework drawing on Big Data to optimize, plan, and manage urban water resources systems (water supply, wastewater, and stormwater systems), and automatically adapt to environmental and policy changes in the face of uncertainty.
Prior to joining the Department of Civil and Environmental Engineering, Prof. Quilty worked as a Research Associate at McGill University where he focused on new AI-based methods for hydrological forecasting. At the same time, he worked as a Water Meter Operations Engineer at the City of Ottawa where he focused on: project/program management, developing water consumption forecasting models for the purpose of establishing water, wastewater, and storm water rates, analysis of water consumption profiles for design, operational, and billing concerns, water meter right sizing, and the development of specifications and standards for water supply system components.
Research Interests
- Hydrological and water resources modelling, forecasting, and simulation
- Data-driven modelling (artificial intelligence, machine learning, deep learning, etc.)
- Data mining
- Time series analysis (e.g., wavelet transforms)
- Big Data applications in hydrology and water resources
- Uncertainty quantification and risk assessment
- Ensemble modelling/forecasting
- Data assimilation
- Drinking water distribution systems
- Optimization
Scholarly Research
My research interests are mainly focused on using novel data-driven (statistical) approaches to address three key issues in hydrological and water resources forecasting: nonlinearity, multiscale change and uncertainty. Most recently I developed a multiscale stochastic data-driven forecasting framework that addresses each of these issues in holistic manner by integrating artificial intelligence/machine learning methods (e.g. neural networks, random forests, etc.) to model nonlinearities; ii) wavelet transforms to handle multiscale change (i.e. trends, periodicities, transients, etc.); and iii) a stochastic 'blueprint' to account for uncertainties in each stage of the hydrological or water resources model design (i.e., input data, input variable selection, parameters, model output, etc.)., I currently serve as an Associate Editor for Journal of Hydrology and have reviewed manuscripts for over a dozen high-impact journals in the water resources domains and beyond, including: Water Resources Research, Journal of Hydrology, Hydrology and Earth System Sciences, Environmental Modeling and Software, IEEE Transactions on Neural Networks and Learning Systems.
Education
- 2018, Doctorate Bioresource Engineering, McGill University, Canada
- 2012, Bachelor's Civil Engineering with Concentration in Management, Carleton University, Canada
Service
- Associate Editor - Journal of Hydrology (2023 - present)
Professional Associations
- Professional Engineers of Ontario
Teaching*
- CIVE 280 - Fluid Mechanics
- Taught in 2020, 2021, 2024
- CIVE 680 - Water Management
- Taught in 2020, 2021, 2023, 2024
- ENVE 224 - Probability and Statistics
- Taught in 2023, 2024
- GEOE 224 - Probability and Statistics
- Taught in 2023, 2024
* Only courses taught in the past 5 years are displayed.
Selected/Recent Publications
- , , , , 0000
- , , , , 0000
- , , , , 0000
- , , , , 0000
- , , , , 0000
- , , , , 0000
Graduate studies
- Currently considering applications from graduate students. A completed online application is required for admission; start the application process now.
- Has Approved Doctoral Dissertation Supervisor (ADDS) status