Research paper
Authors:
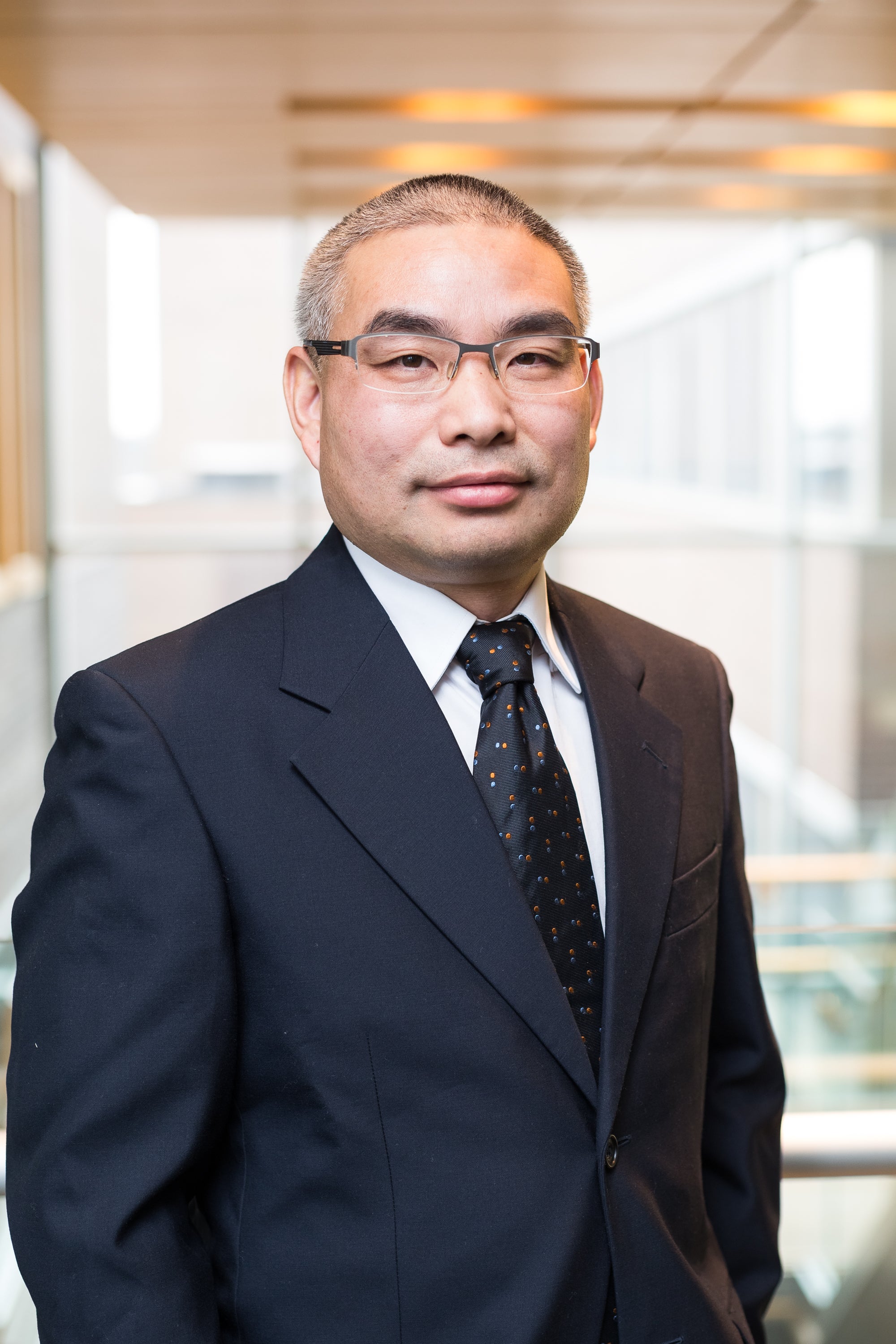
Alan Huang, University of Waterloo, School of Accounting and Finance (pictured)
Zijia Du, Shanghai Jiao Tong University (SJTU), Antai College of Economics and Management, Students
Russ Wermers, University of Maryland - Robert H. Smith School of Business; European Corporate Governance Institute (ECGI)
Wenfeng Wu, Shanghai Jiao Tong University - Antai College of Economics & Management
We use Word2vec to develop a financial sentiment dictionary from 3.1 million Chinese-language financial news articles. Our dictionary maps semantically similar words to a subset of human-expert generated financial sentiment words. In validation tests, our dictionary scores the sentiment of articles consistently with human reading of full articles. In return association tests, our dictionary outperforms and subsumes previous Chinese financial sentiment dictionaries, such as direct translations of Loughran and McDonald’s (2011) English-language financial dictionary. We also generate a list of politically-related positive words that is unique to China; we find that this list has a weaker association with returns than does the list of other positive words. We demonstrate that state media uses more politically-related positive and fewer negative words, and exhibits a sentiment bias. This bias renders the state media’s sentiment as less return-informative. Our findings demonstrate that dictionary-based sentiment analysis exhibits strong language and domain specificity.