Revolutionizing Forest Management with AI
PhD Candidate Lanying Wang is supporting sustainable resource management using remote sensing and advanced technology.
Estimated reading time: 1:49
Forests play a critical role in climate change adaptation due to their ability to trap and store carbon. To determine a forest’s capacity for carbon sequestration, it is important to inventory and monitor forested areas regularly. Tree species classification is a vital component of forest management and can assist with calculating carbon sequestration potential.
In-person monitoring of forests can be difficult, especially in remote locations or large areas. Remote sensing techniques have been proven effective at assisting with forest management, notably LiDAR (Light Detection and Ranging). LiDAR uses a pulsed laser to measure ranges, which results in a three-dimensional point cloud that can be used for data analysis. When LiDAR data is collected over a large area with an aircraft operating at a high elevation, the density of the point cloud can be sparse. These datasets can be difficult to conduct accurate individual tree-level species classification.
Lanying Wang, a PhD candidate in the Department of Geography and Environmental Management, is combining remotely sensed data and deep learning (DL) models to improve data accuracy and applicability.
“Deep learning models are like digital brains that learn patterns from large amount of data” Wang explains. “In forest inventory, they can analyze remotely sensed data to identify species and extract attributes such as height and crown size.”
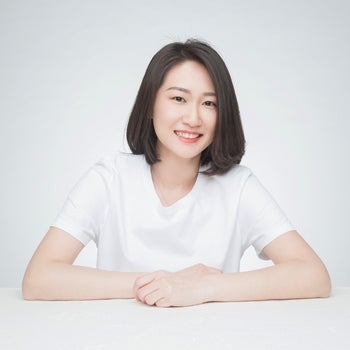
Deep learning models are like digital brains that learn patterns from large amount of data.
In a recent study, Wang developed and evaluated a DL model specifically designed to improve classification accuracy of tree species. Using a low density point cloud from a forest in York Region, Ontario, Wang trained the model using a representative sample set, ensuring it could generalize to all scenarios and enable accurate predictions. To evaluate accuracy, the data was compared to field data collected on site.
The results showed Wang’s DL model not only enhances classification accuracy but also reduces the need for extensive training data, making it more reliable for tree specific classification than previous methods. This work enhances the understanding of tree species classification from low-density point clouds and adds to the growing development and application of deep learning in forestry sciences.
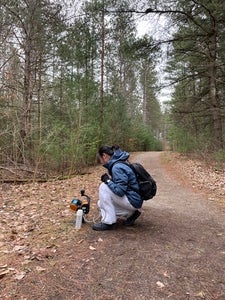
“AI and deep learning will transform forest inventory tasks to be more accurate, scalable, and accessible, supporting sustainable resource management,” she confirms.
In the future, Wang plans to expand her work to create an improved workflow that integrates both segmentation and classification processes for individual tree-level inventory.
The research, Individual tree species classification using low-density airborne multispectral LiDAR data via attribute-aware cross-branch transformer, authored by Wang and others, was recently published in Remote Sensing of Environment.