Research interests: Process Data Analytics; Machine Learning; Statistical Process Monitoring and Fault Diagnosis; Optimization and Control of Process and Energy Systems; Process Systems Engineering
Biography
Professor Qinqin Zhu is an assistant professor in the Department of Chemical Engineering at the University of Waterloo. She is also a faculty member in the Waterloo Institute for Nanotechnology (WIN), Waterloo Artificial Intelligence Institute (Waterloo.AI) and Waterloo Institute for Sustainable Energy (WISE). Professor Zhu received her PhD degree from the Chemical Engineering department at the University of Southern California. Prior to UWaterloo, She worked as a senior research scientist at Facebook Inc. in the United States.
Education
- Doctoral Degree, Chemical Engineering, University of Southern California, 2017
- Master's Degree, Computer Science, University of Southern California, 2016
- Bachelor's Degree, Automation, Zhejiang University, 2013
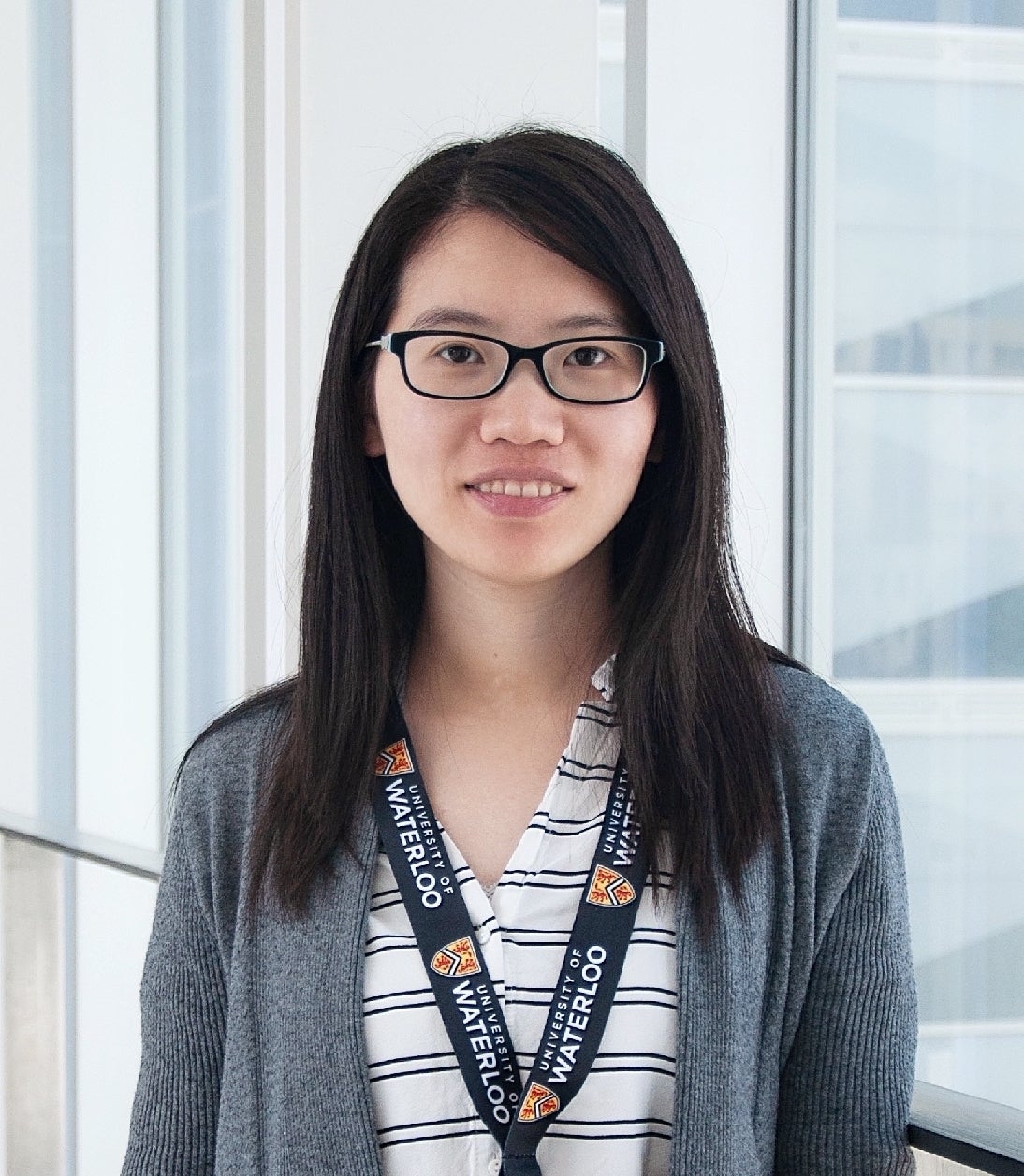