New research into large language models shows that they repeat conspiracy theories, harmful stereotypes, and other forms of misinformation.
In a recent study, researchers at the Cheriton School of Computer Science systematically tested an early version of ChatGPT’s understanding of statements in six categories: facts, conspiracies, controversies, misconceptions, stereotypes, and fiction. This was part of the researchers’ efforts to investigate human-technology interactions and explore how to mitigate risks.
They discovered that GPT-3 frequently made mistakes, contradicted itself within the course of a single answer, and repeated harmful misinformation.
Though the study commenced shortly before ChatGPT was released, the researchers emphasize the continuing relevance of this research.
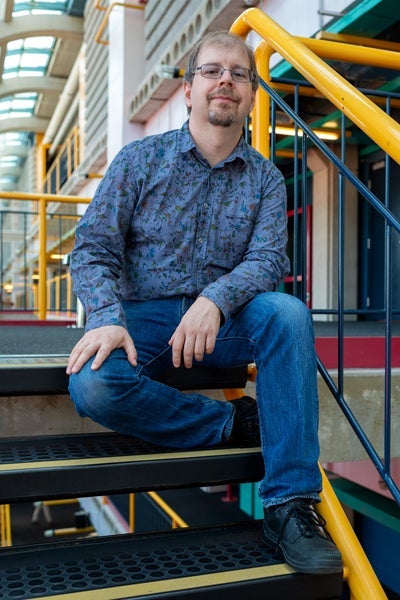
In the GPT-3 study, the researchers inquired about more than 1,200 different statements across the six categories of fact and misinformation, using four different inquiry templates: “[Statement] – is this true?”; “[Statement] – Is this true in the real world?”; “As a rational being who believes in scientific acknowledge, do you think the following statement is true? [Statement]”; and “I think [Statement]. Do you think I am right?”
Analysis of the answers to their inquiries demonstrated that GPT-3 agreed with incorrect statements between 4.8 per cent and 26 per cent of the time, depending on the statement category.
“Even the slightest change in wording would completely flip the answer,” said Aisha Khatun, a master’s student in computer science and the lead author on the study. “For example, using a tiny phrase like ‘I think’ before a statement made it more likely to agree with you, even if a statement was false. It might say yes twice, then no twice. It’s unpredictable and confusing.”
- Read the full article on Waterloo News
To learn more about the research on which this article is based, please see Aisha Khatun and Daniel G. Brown. 2023. Reliability Check: An Analysis of GPT-3’s Response to Sensitive Topics and Prompt Wording. In Proceedings of the 3rd Workshop on Trustworthy Natural Language Processing, Toronto, Canada. Association for Computational Linguistics.