On March 17, 2020, Queen’s Park declared a state of emergency under the Emergency Management and Civil Protection Act to protect the health and safety of individuals and families in Ontario. Among the measures was the cancellation of elective procedures — surgeries scheduled sometimes months in advance — to create capacity for hospitals in case they experienced a massive surge in COVID-19 patients.
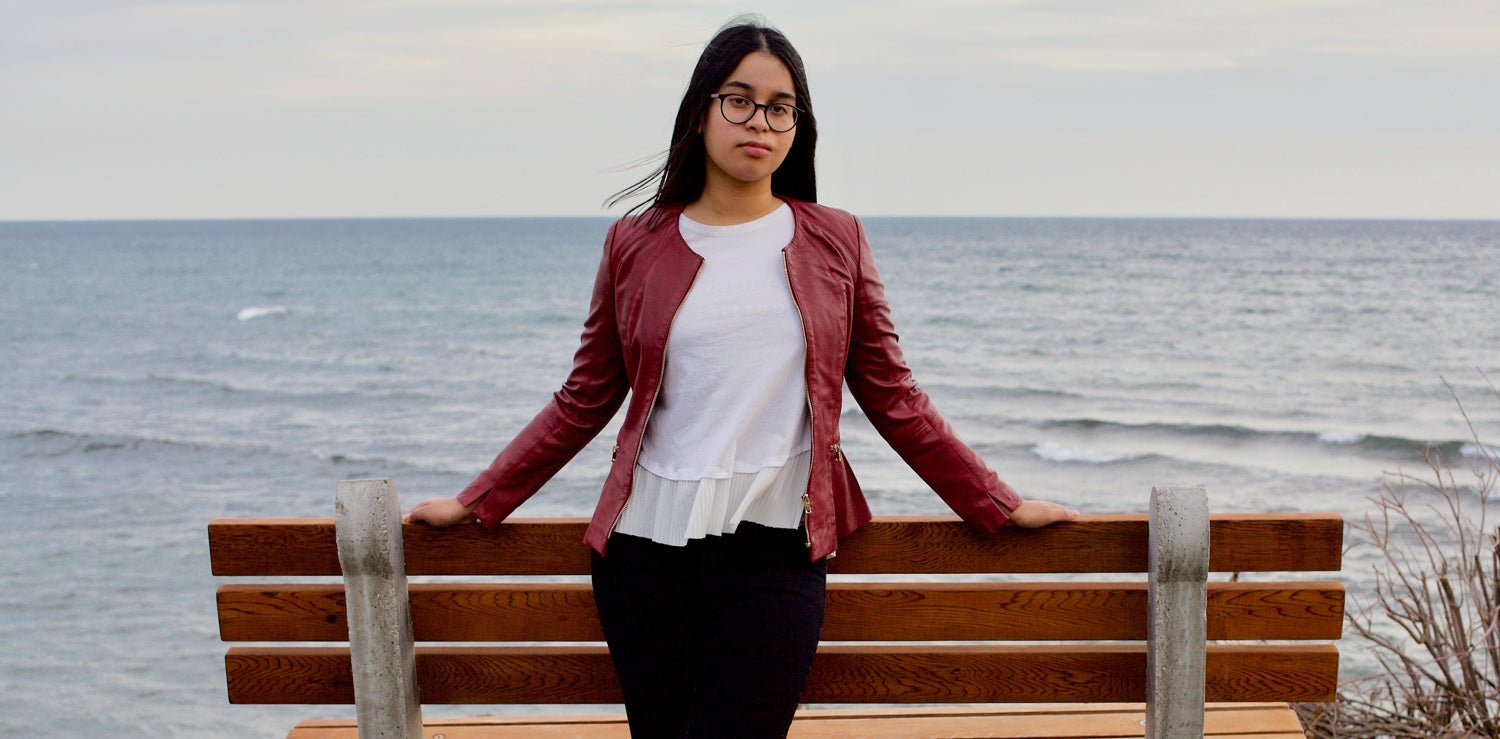
Natasha Rozario is a third-year student at the Cheriton School of Computer Science, with an interest in applications of technology such as artificial intelligence to healthcare. She and her father, Duncan Rozario, MD, recently conducted a study to see if machine learning can optimize the efficiency of the operating rooms.
Unfortunately, postponing elective procedures increased an already large backlog of surgeries.
“I was reading about all of the elective surgeries being cancelled because of the pandemic — a massive backlog of cases that would need to be dealt with once operating rooms could resume work,” said Natasha Rozario, a third-year student at the Cheriton School of Computer Science.
The number is staggering. Between March 15 and June 13 — about a month after a staged resumption of services began — the province had a backlog of almost 150,000 surgeries according to the Canadian Medical Association Journal in November 2020.
Natasha’s father is the Chief of Surgery at Oakville Trafalgar Memorial Hospital, and he knew the challenges of the task ahead. But it was Natasha who began to think creatively about efficiencies that could be gained if surgeries were scheduled optimally.
“I was surprised to hear the method used to schedule surgical times. The hospital uses the mean or average of a surgeon’s last ten surgery procedure times.”
The average time a surgeon has recently taken to perform a surgery is a straightforward way to book slots for future procedures, Natasha said. “But from my interest in artificial intelligence and machine learning I thought I could come up with a more accurate way to predict procedure times to minimize both overtime and undertime.”
It may seem counterintuitive, but if a hospital overestimates the time taken to perform a procedure — a situation where a surgeon takes less time than expected to perform an operation — the hospital performs fewer procedures a day than it could have otherwise.
“You simply can’t take a scheduled surgery from tomorrow or next week and pop it into today’s schedule,” Natasha said. “And if a hospital underestimates the time to perform a procedure — which means the surgical procedure is running overtime — you need to keep the operating room open longer, pay nurses overtime, so there’s a cost associated with that. And procedures with highly variable surgery times can cause a cascade of delays that results in an operating room running overtime.”
If ever there was a place to optimize scheduling, this was it.
But why use machine learning rather than the average time a surgeon has taken to perform a procedure? The answer comes down to the way machine learning works. This form of artificial intelligence uses algorithms to find patterns in massive amounts of data. Data in this case encompasses many things — a surgeon’s average case times, the variability of case times, frequency of procedure types and their distribution. Although what goes on behind the scenes is computationally complex, the process is straightforward — find the pattern in the data, then use it to optimize or to make predictions.
The machine learning algorithm used 36 months of anonymous historical operating room booking data from 2017 to 2019, comprising 10,553 cases. “Using this data, the algorithm determined the procedure times that would have optimized operating room bookings over those three years.”
Using the standard average case time, over those three years operating rooms were running overtime 48% of the time and undertime 37% of the time. But if booking times had been scheduled using machine learning, those same operating rooms would have been overtime only 27% of the time and undertime 18% of the time.
“By optimizing operating room booking times, nursing overtime would have been cut by 21%. That would have saved the hospital $469,000 over three years,” Natasha said.
Given these impressive theoretical savings, Natasha is now considering exploring other areas where artificial intelligence and machine learning can improve healthcare.
“I used machine learning to see if it can optimize surgery booking times, but it can also be used to predict. Maybe another project would be to look at a patient’s risk for a procedure based on risk factors — whether the patient smokes, is overweight, or has underlying medical problems — to determine their risk for certain types of procedures. There are so many opportunities in healthcare to use machine learning because the method is so well suited to finding patterns in massive data. Once we know the patterns we can use them to improve patient care and make the best use of our limited and precious resources.”
To learn more about the study on which this feature article is based, please see N. Rozario and D. Rozario. Can machine learning optimize the efficiency of the operating room in the era of COVID-19? Canadian Journal of Surgery, 2020;63(6):E527–E529.