Professor Florian Kerschbaum has been awarded $2 million through the Ontario Research Fund to develop innovative data science and machine learning techniques aimed at safeguarding Ontario’s financial technology and manufacturing sectors from inadvertent data leaks. The project, led by Professor Kerschbaum as principal investigator and Professor N. Asokan as co-principal investigator, will enhance data security in these critical industries.
“By investing in cutting-edge research, we are safeguarding Ontario’s position at the forefront of innovation that continues to be competitive on a global scale and has the ability to attract the best and brightest talent to our province,” said Jill Dunlop, Minister of Colleges and Universities. “This will help ensure the social and economic opportunities that result from discoveries made in Ontario benefit Ontarians and the Ontario economy.”
ORF contributes up to one-third of the total project funding, with the remaining funds provided by private sector and institutional partners. Professor Kerschbaum’s research is one of four ORF-supported projects, totaling nearly $8 million, allocated to Waterloo researchers.
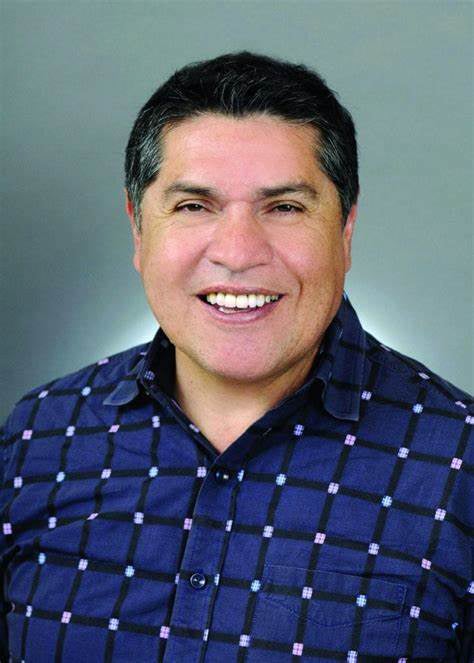
“At RBC, we value the privacy and security of our clients above all and throughout the years, we’ve had the opportunity to develop and help support initiatives that enhance and innovate on what’s possible in these critical areas of cyber safety,” said Eddy Ortiz, VP, Solution Acceleration & Innovation at RBC (inset on right). “As a result, we’ve been able to successfully launch numerous privacy-preserving patents, publications and products, including our virtual clean room, Arxis. We are also proud to work and collaborate with some of the leading laboratories in the world, including University of Waterloo, in the area of federated learning.”
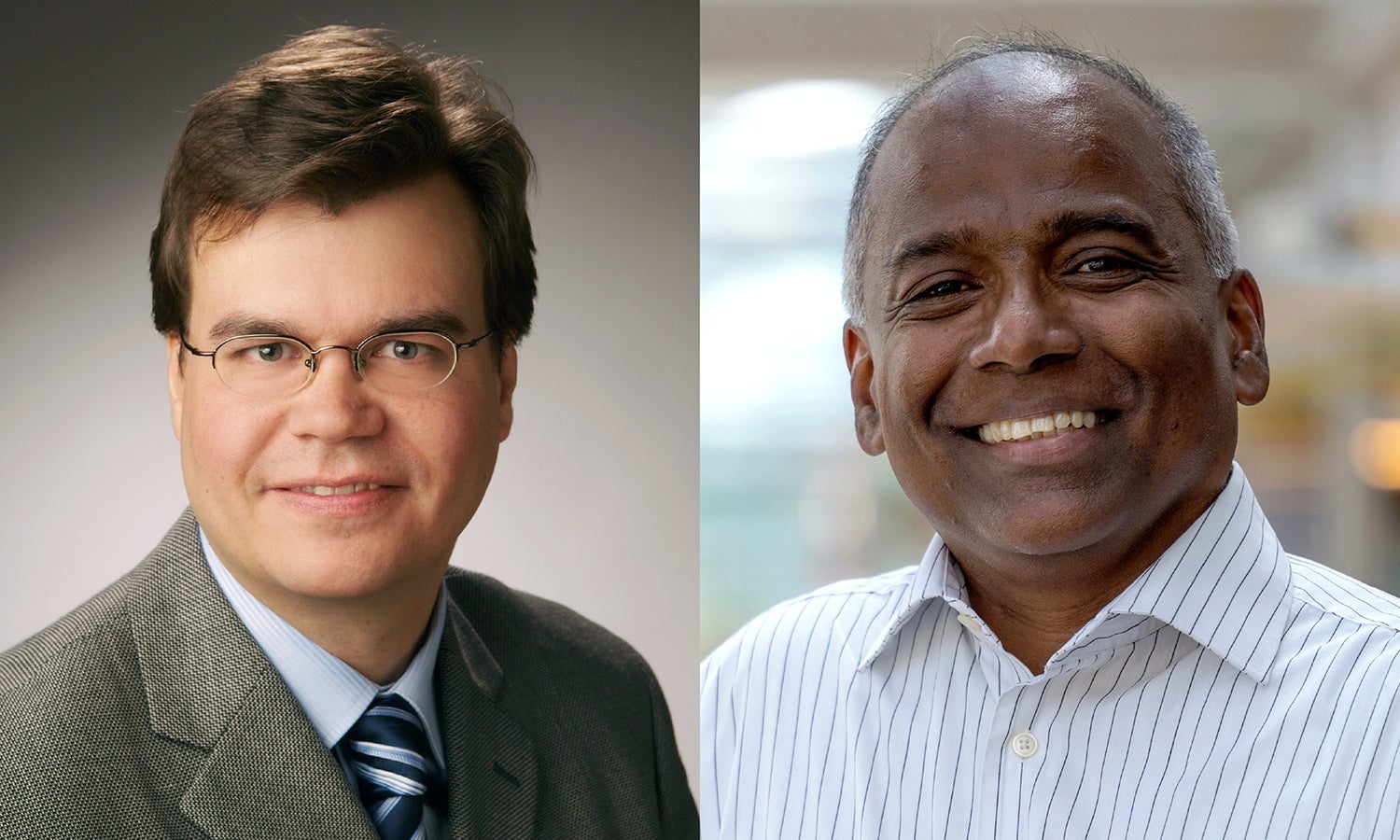
L
to
R:
Professors
Florian
Kerschbaum
and
N.
Asokan
Professor
Kerschbaum
is
recognized
internationally
for
his
expertise
in
data
security.
In
2019,
he
was
named
the
NSERC/RBC
Industrial
Research
Chair
in
Data
Security,
the
same
year
he
became
an
ACM
Distinguished
Member
as
well
as
recognized
by
CS-Can
|
Info-Can
as
an
Outstanding
Young
Computer
Scientist.
In
2020,
he
became
a
Fellow
of
the
Balsillie
School
of
International
Affairs
and
in
2022
received
the
Faculty
of
Mathematics
Golden
Jubilee
Research
Excellence
Award.
Professor
Asokan
joined
the
Cheriton
School
of
Computer
Science
in
September
2019
as
a
Cheriton
Chair,
an
endowed
position
supported
by
the
David
R.
Cheriton
Endowment
for
Excellence
in
Computer
Science.
He
is
a
principal
investigator
at
the
Private
AI
Collaborative
Research
Institute,
a
research
group
funded
jointly
by
Intel
Labs
and
other
industry
partners
to
develop
fundamental
technologies
that
are
instrumental
in
strengthening
the
security,
privacy
and
trustworthiness
of
decentralized
artificial
intelligence.
In
2018,
he
was
named
a
Fellow
of
ACM
and,
in
2023,
a
Fellow
of
the
Royal
Society
of
Canada.
He
is
also
the
Director
of
the Cybersecurity
and
Privacy
Institute.
About the research
Professor Kerschbaum’s research will address the growing demand for data collection in the digital economy while ensuring robust data privacy protections. Protecting data privacy is essential to prevent accidental leaks of private information. Although data science involves intentionally releasing some information, privacy-preserving techniques — such as those provided by cryptography and statistical guarantees — can limit what can be inferred about private data. As such, systems designed for privacy-preserving data science must balance intentional leakage against the risk of unintentional leakage, while being computationally efficient.
“Our project aims to address this trade-off in utility, privacy and efficiency in private computation by taking a holistic approach to data science,” said Professor Kerschbaum. “The goal is to achieve better trade-offs that benefit Ontario’s fintech and manufacturing industries by supporting their business goals while preserving privacy for both their customers and businesses. As the project has many components, it will also result in training dozens of students at both undergraduate and graduate levels over five years, providing them with the knowledge and expertise for careers in privacy-preserving data science.”