Freda Shi joined the Cheriton School of Computer Science as a tenure-track Assistant Professor in July 2024. In September 2024 she was named a CIFAR AI Chair and a faculty member at the Vector Institute.
Professor Shi’s research interests are in computational linguistics and natural language processing. She works towards deeper understandings of natural language and the human language processing mechanism, as well as how these insights can inform the design of more efficient, effective, safe and trustworthy NLP systems. She is particularly interested in learning language through grounding, computational multilingualism, and related machine learning aspects.
Her representative work includes grounded syntax and semantics learners, the contextualized bilingual lexicon inducer, and the substructure-based zero-shot cross-lingual dependency parser. She has also worked on searching for evidence of semantics encoded in large language models. Her work has been recognized with best paper nominations at ACL 2019 and 2021, and was supported by a Google PhD Fellowship. She has a PhD in computer science from Toyota Technological Institute at Chicago and a BS in Intelligence Science and Technology from Peking University.
She leads the Computation, Language, Intelligence, and Grounding (CompLING) Lab, a group of researchers who work towards understanding human language with the help of computational methods. As of January 2025, her work has been cited more than 2,750 times with an h-index of 14 according to Google Scholar.
What follows is a lightly edited transcript of a conversation with Professor Shi, where she shares insights into her research, advice for aspiring computer scientists, and her enthusiasm for joining the Cheriton School of Computer Science.
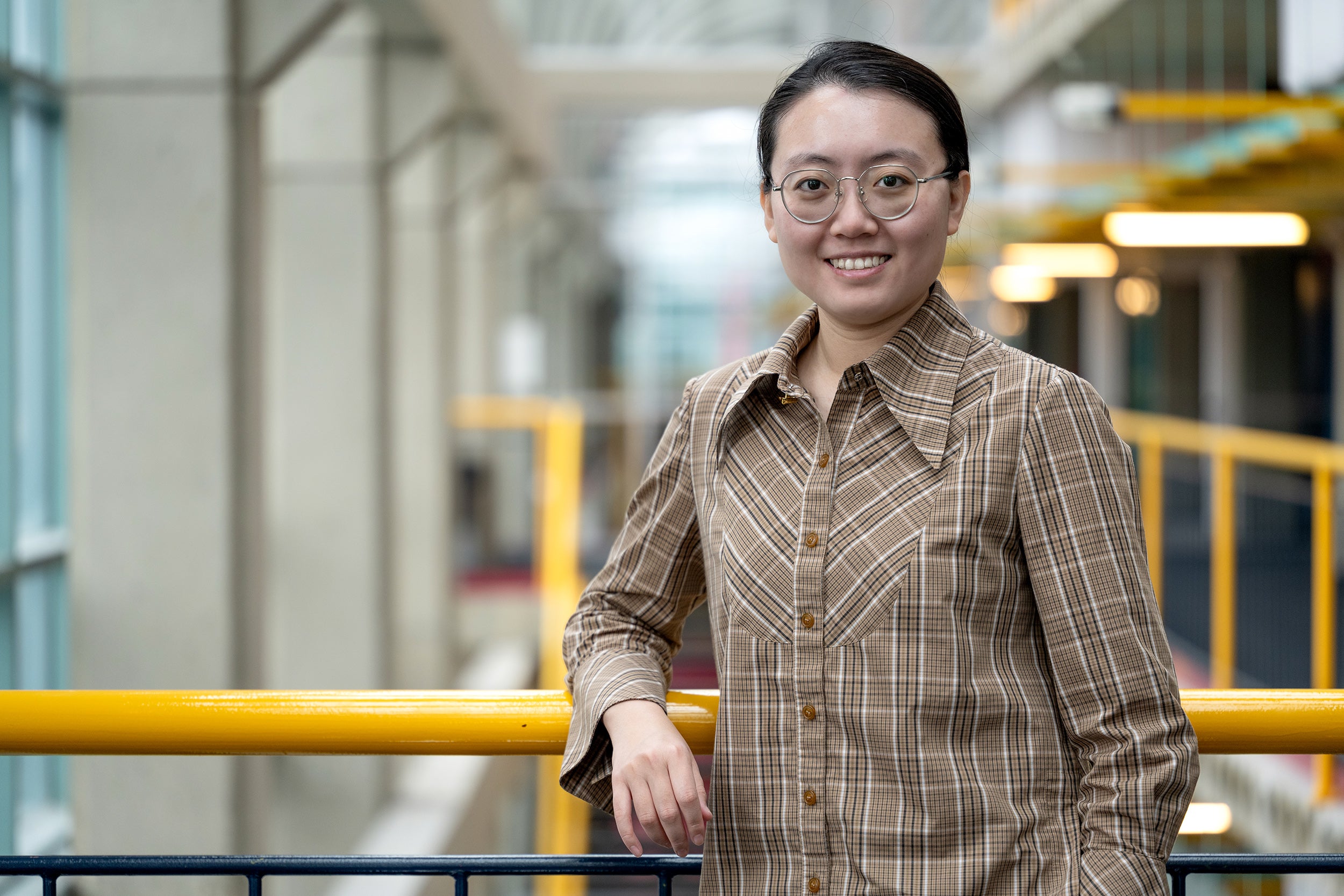
Tell us a bit about your research.
My research is at the intersection of computational linguistics and natural language processing and related aspects in machine learning. I use language models and computational techniques to study linguistics and I use linguistic insights to improve the performance and interpretability of language models. My work informs both linguistics and computational methods.
Recently, my research has extended to computational psycholinguistics. This involves collecting human behavioral data, using computational tools to analyze it, and rigorously comparing large language models to human cognition. My goal is to explore the potential of using these models as human mental models.
What do you see as your most significant contribution?
I would say that my most significant contribution is my study from 2019 that combined syntax, a linguistic concept, with visual grounding, which involves connecting language to the real physical world. Before this work, researchers mostly used predefined syntax to extract some phrases and find their visual cues in the corresponding visual data. In my work, I proposed to induce the syntactic structures based on the parallel image-text captions. I showed that there are some meaningful and reasonable connections between syntax and visually grounded semantics. Visually grounded semantics is a long-standing hypothesis that had never been verified before that.
What challenges in computational linguistics do you find most interesting to tackle?
Cognitive scientists are interested in using models — transformers and ChatGPT-style models — to serve as human predictors or as human mental models. One challenge is determining how these models are similar or different from human mental models. This is still an open question and designing a novel and rigorous metric to evaluate it from this perspective would be interesting and informative.
For computational linguistics, there are a lot of scattered challenges, for example how languages evolve. We can’t do fully controlled experiments, but to some extent we can as we have computational agents and can simulate based on our understanding of human language. We can build agents to simulate human behaviours and observe what happens in communications between the agents. That wouldn’t provide a fully reliable explanation of language evolution, but it might shed light on how language evolves.
What advice would you give to students interested in pursuing research in your area?
First, I would say avoid being distracted by whatever is the latest research trend. There are many fundamental problems that have not been solved, such as the interpretability of the language models. That’s unlikely to be solved within the next few years. New techniques will emerge but it’s important to stay focused on the bigger picture. Researchers have been struggling with interpretability within neural networks for more than a decade.
Second, always pay close attention to your data, as it can reveal crucial insights that debugging alone may not uncover. Looking at the data and the encoding mechanism may reveal something to examine further. For example, I trained a cross-lingual dependency parser and it performed very well except on one language. I looked more closely at the data and discovered the issue — that the data had some bizarre encodings.
So, if you look carefully at the data some issues will become apparent as will ways to solve them. It’s much more important to look at your data than I had originally appreciated. In computer science we often have tonnes of data, which can be a huge asset. But we also have so much data that we may not look at it carefully.
Do you see opportunities for collaborative research at the Cheriton School of Computer Science?
I’m exciting to collaborate with members of the core natural language processing group at the School — Victor Zhong, Jimmy Lin, Wenhu Chen and Yuntian Deng. They are an exceptionally strong group of colleagues. They are more focused on NLP and machine learning whereas I’m more focused on theoretical linguistics, but we are working in a similar area and can learn from each other.
Beyond the NLP group, I see potential to collaborate with the larger machine learning group. I am interested in interpretability issues of large language models and this group would have valuable insights into it.
I am also eager to work with my colleagues Pascal Poupart and Jesse Hoey in artificial intelligence. I’m co-advising a student with Dan Brown, who works on computational creativity, and I will be co-advising a student with Robin Cohen, who’s been a very supportive faculty mentor.
What aspect of joining the Cheriton School of Computer Science excites you the most?
The School has a strong tradition of excellence in computer science, with supportive colleagues and highly motivated students, both undergraduate and graduate. I’m working with three undergraduate research assistants and they are all exceptionally strong. Even though they are undergrads they approach research with the rigour of a good graduate student.
On a personal level, joining the School has been a bit like a family reunion. Some of my relatives settled in the Waterloo region while I was completing my PhD at the Toyota Technological Institute at Chicago, so this feels like coming home to be here. Additionally, several of my friends graduated from the University of Waterloo, so it’s great to now teach the next generation of students.
Who has inspired you in your career?
Ludwig Wittgenstein has been one of my greatest inspirations. He passed away quite a few years before I was born so I never met him, but when reading his biography and his work on the philosophy of language I knew I wanted to work at the intersection of computer science and linguistics.
Wittgenstein talked about a lot of interesting problems at the intersection of philosophy and language. He is the person who bridged the gap between linguistics and philosophy, and he raised a lot of interesting questions at the boundary of meaning and related aspects of language. Before Wittgenstein, people thought of language more as a toolkit.
During my undergrad degree at Peking University I majored in computer science with a minor in sociology, and Wittgenstein’s work inspired me to pursue what I am now studying in my career as a professor.
I was also fortunate to have outstanding mentors during my PhD. My co-advisors — Kevin Gimpel and Karen Livescu — were hugely inspiring. Both are solid, dedicated scientists and I was fortunate to be advised by them. And during the last two terms of my PhD I was a visiting student in Roger Levy’s group at MIT. He has been an inspiration to me to pursue research at the intersection of psycholinguistics and computational methods. He’s a leader in this field.
What do you do in your spare time?
I’m learning to snowboard. My husband is a good snowboarder and we wanted to find an activity we could do together in the winter. I grew up in a part of China that gets snow but we don’t have a tradition of snowboarding as the region is not mountainous.
I’d also like to try cross-country skiing and snowshoeing. Maybe it’s best to start with safer winter sports.