Professors Olga Veksler and Yuri Boykov joined the David R. Cheriton School of Computer Science earlier this year. Previously, both were full professors in the Department of Computer Science at Western University, where they were faculty members for 14 years.
Their research interests are in the area of computer vision. In particular, Olga’s interests are in visual correspondence and image segmentation, and Yuri’s also include 3D reconstruction and biomedical image analysis.
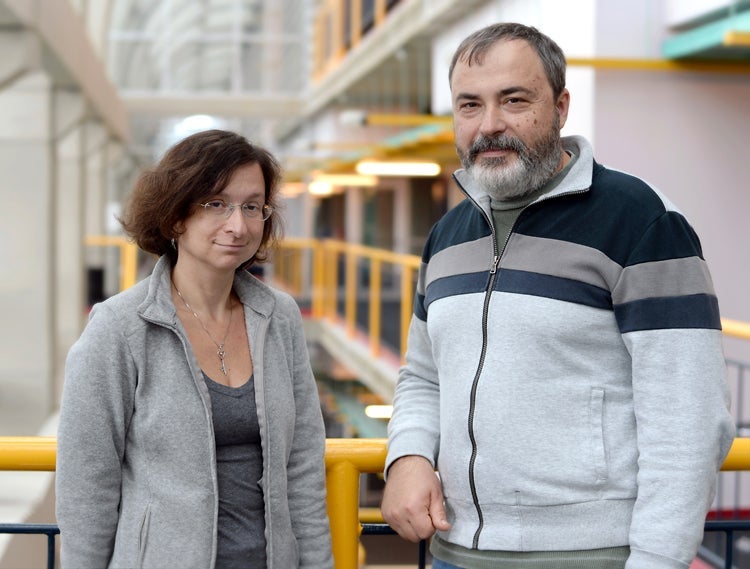
What attracted you to the Cheriton School of Computer Science?
OV: The school has an excellent reputation in general and very much so among computer scientists, and the students here are exceptionally strong.
YB: Yes, all that and the University of Waterloo also has a recent Nobel Prize winner in physics.
Tell us about your computer vision research.
OV: Computer vision in general is about analyzing images and video. The images can come from a large variety of sources. People often think of photographs, but images can also come from devices like ultrasound and X-ray machines or MRI scanners.
When a human looks at a photograph, he or she can understand what’s in the image, what the person in the image is doing, maybe even predict what’s going to happen next. When computers look at images, they often struggle with these same tasks. Computer vision may seem easy because humans do it so effortlessly, but it’s challenging task for a computer to do well.
When given an image, we try to get the computer to understand what the objects in them are — where’s the person, where’s the car, where’s the building. We have also worked quite a bit on understanding how far away objects are. Humans are very good at understanding the world in three dimensions because we have two eyes and a brain that let us perceive the world in 3D.
We can get computer to do the same thing using stereo images. If you use two cameras, a computer can use the images to calculate how far away an object is. We have also worked on motion — when an object moves in a scene, where is it going, what’s its trajectory.
Our approach has been very mathematical — solving optimization problems using various optimization methods. Many tasks that are natural and easy to do for humans — figuring out where objects are, where they’re going, or how far they are away — can be solved computationally using beautiful mathematical optimization models.
YB: The kind of image analysis I work on is both similar to and different from Olga’s. For example, my work includes medical image analysis, which is something humans don’t do easily or naturally, at least not without years of training and study.
Medical images are very different from photographs for us. Radiologists need years of training to understand and interpret what they see. But the task is similar to what Olga does in the sense that we’re trying to get computers to understand and analyze images. For computers, analyzing images is difficult, just as it for humans when they first look at medical images.
OV: Computer vision is part of artificial intelligence in general, and machine learning methods are being used more and more now. It used to be that computer vision was about one-third machine learning. Now, it’s around 90% machine learning and that has come about in just the last few years.
Do you see opportunities for new, collaborative research, given the breadth and depth of research conducted at the school?
YB: The machine learning group here at the school is much bigger than at Western and it’s more established, so, yes, there’s lots of potential for collaboration. However, the school hasn’t conducted a lot of research specifically in computer vision lately, so we’re helping to address that gap.
What do you consider your most significant contribution in computer vision?
OV: We have a paper about optimization with graph cuts that has won multiple awards. A lot of conferences have best paper awards and some have categories like best paper ten years after. Those kinds of papers are considered works that have withstood the test of time.
YB: Our paper — Fast approximate energy minimization via graph cuts — which was published in IEEE Transactions on Pattern Analysis and Machine Intelligence, won a test-of-time award at ICCV 2011, the International Conference on Computer Vision. This is the top conference in computer vision.
The optimization code based on that work typically is downloaded 20 to 30 times a day. That paper is our highest cited paper.
OV: This research is now a feature in the latest version of Microsoft Office Suite. The background removing tool is based on the work in that paper.
YB: The technologies for that belonged to Cornell and Siemens, where I worked for some time, but since then Microsoft, Adobe, Google bought or shared the patent — some of them have patent sharing agreements.
The technology works well to remove backgrounds in two dimensional images, but it works even more impressively with 3D data. This is very powerful when used with 3D medical images.
How are you finding life at Waterloo?
OV: We both like smaller cities and we both like to walk, so living here is great. We can walk to work.
YB: We have to walk because we haven’t bought a parking permit yet. [laughs] But, yes, we like to walk.
OV: We have a daughter in high school and a son attending university here at Waterloo. We’re all here.
YB: We also both love music. I used to play the piano a bit but it’s been a few years.
OV: We used to drive to from London to hear the concerts at Kitchener-Waterloo Chamber Society on Young Street near the park. It’s an excellent venue with many chamber music concerts every week. I guess we can walk to the performances now. [laughs]
YB: It’s really a quality-of-life issue. We like the community, we like the university, we enjoy walking, and we like that the city has built a tramline. This is a good place for us.