Since the COVID-19 pandemic began, researchers and clinicians have rushed to understand the available treatments and procedures to mitigate this rapidly growing threat to human health. The sheer volume of studies published on COVID-19 — in countries spanning the globe — as well as lessons learned from prior epidemics and pandemics, simply cannot be gathered and assessed quickly enough using traditional manual methods during this time of crisis.
The urgency of the COVID-19 pandemic has necessitated a transformation in interdisciplinary collaboration, as well as in the process for completing systematic reviews for evidence-based medicine, so that reviews that used to take researchers many months to complete can now accomplished in a matter of days or weeks.
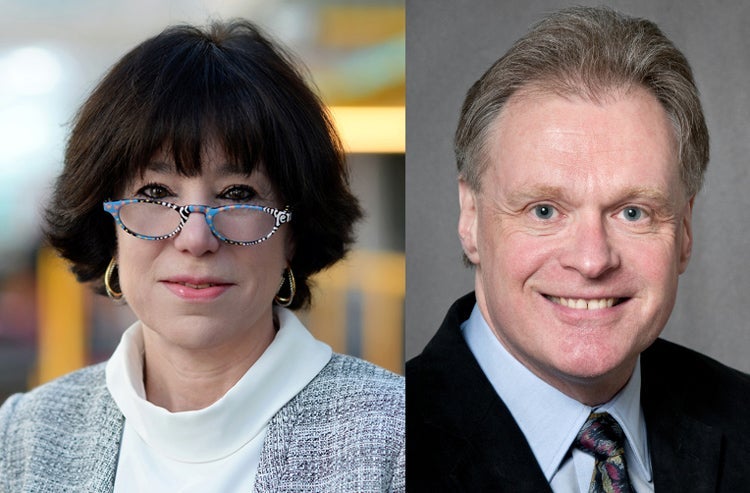
Maura
R.
Grossman
is
a
Research
Professor
and
Director
of
Women
in
Computer
Science
at
the
Cheriton
School
of
Computer
Science,
and
an
Adjunct
Professor
at
Osgoode
Hall
Law
School,
as
well
as
an
eDiscovery
attorney
and
consultant
in
Buffalo,
New
York.
Gordon
V.
Cormack
is
a
Professor
at
the
Cheriton
School
of
Computer
Science. His
primary
research
focus
is
on
high-stakes
information
retrieval.
To guide decisions by healthcare providers on the frontlines of the healthcare crisis, Cheriton School of Computer Science Professors Maura R. Grossman and Gordon V. Cormack have been working with the knowledge synthesis team at St. Michael’s Hospital in Toronto, on behalf of the Canadian Frailty Network and Health Canada, to automate these literature searches.
The goal of their efforts is to help the team to quickly identify clinical studies that have evaluated the effectiveness and safety of various measures to keep nursing care facilities safe, as well as treatments for patients with COVID-19. The results of these systematic reviews are then used to support treatment and policy decisions. Importantly, new evidence must be interpreted in light of the lessons learned from studies conducted during previous epidemics and pandemics, such as Severe Acute Respiratory Syndrome (SARS), which caused a disease outbreak beginning in 2002, and Middle East Respiratory Syndrome (MERS), which caused a disease outbreak beginning in 2012.
Using Continuous Active Learning (CAL) Technology-Assisted Review (TAR) — a supervised machine learning approach that Professors Grossman and Cormack originally developed to expedite the review of documents in high-stakes legal cases — they have now applied this same method to automate literature searches in massive databases containing health-related studies for systematic reviews.
Systematic reviews use formal explicit methods — in other words, pre-specifications — that set forth precisely what question is to be answered, how evidence is to be searched for and assessed, and how the information will be synthesized to reach a conclusion. Conducting a systematic review involves multiple processes typically requiring many tedious steps and numerous hours of expert labour. An average systematic review can take a team of researchers a year or more to produce. In this time of emergency, Professors Grossman and Cormack and the St. Michael’s researchers have combined AI technology with a larger review team to produce systematic reviews in about two weeks.
“Our CAL tool identifies for screening the abstracts or studies most likely to meet the specific inclusion criteria, based on studies that have previously been identified and meet the inclusion criteria,” Professor Cormack explained. “This process continues iteratively until none of the identified studies appear to meet the inclusion criteria.” Medical researchers then review the studies identified by the CAL tool for inclusion or exclusion. “CAL is able to identify potential studies for inclusion more quickly and more accurately than traditional methods,” noted Professor Cormack.
“Searching and finding studies for systematic reviews has traditionally been a time-consuming and laborious process that uses keyword search, followed by manual screening of abstracts, and finally full papers,” Professor Grossman added. “We are instead training a machine learning algorithm to perform the initial steps in this task. We use the trained algorithm to search multiple, massive medical and scientific databases, in different languages, in real-time, as they are updated. We then send to the research team at St. Michael’s the papers they need to consider for inclusion in their systematic reviews. Our goal is to find as many as we can, as quickly as we can, so that researchers, clinicians, and public health officials can quickly draw sound conclusions about the treatments and other procedures that are likely to be the most promising, and to dismiss those that are not.”
The systematic reviews made possible by this work
-
Preventing
the
transmission
of
Coronavirus
(COVID-19)
in
older
adults
aged
60
years
and
above
living
in
long
term
care:
A
rapid
review
Prepared for The Canadian Frailty Network -
Rapid
scoping
review
of
the
effectiveness
and
safety
of
therapeutic
options
for
COVID-19
and
other
coronaviruses
that
cause
serious
respiratory
infections
Prepared for Health Canada
Media coverage and articles about this work
-
Maura
Grossman
and
Gordon
Cormack
put
TAR
to
work
to
help
with
the
COVID-19
fight:
eDiscovery
Trends
eDiscoveryToday, Doug Austin, May 7, 2020 -
A
world
of
difference:
Technology-assisted
review
in
the
fight
against
COVID-19
Complex Discovery, Rob Robinson, May 7, 2020 -
Can
technology-assisted
review
expedite
finding
the
cure
for
COVID-19?
Epiq Angle, May 20, 2020 -
Can
technology-assisted
review
expedite
finding
the
cure
for
COVID-19?
JD Supra, May 21, 2020 -
Staying on top of COVID-19 discoveries
The Waterloo Region Record, Robert Williams, May 24, 2020 -
AI helping physicians, policy makers get the COVID-19 information they need
Georgetown University Law Center, Executive and Continuing Legal Education -
How E-Discovery Software Is Helping Battle COVID-19
Above the Law, Robert Ambrogi, June 29, 2020 -
The Battle Against COVID-19: How eDiscovery Software is Playing a Role
Legal Talk Network, Sharon Nelson and John Simek welcome lawyer and Research Professor Maura Grossman, July 16, 2020 -
The Battle Against COVID-19: How E-Discovery Software is Playing a Role
Ride The Lightning: Electronic Evidence and Information Security Blog, Sharon D. Nelson, July 23, 2020