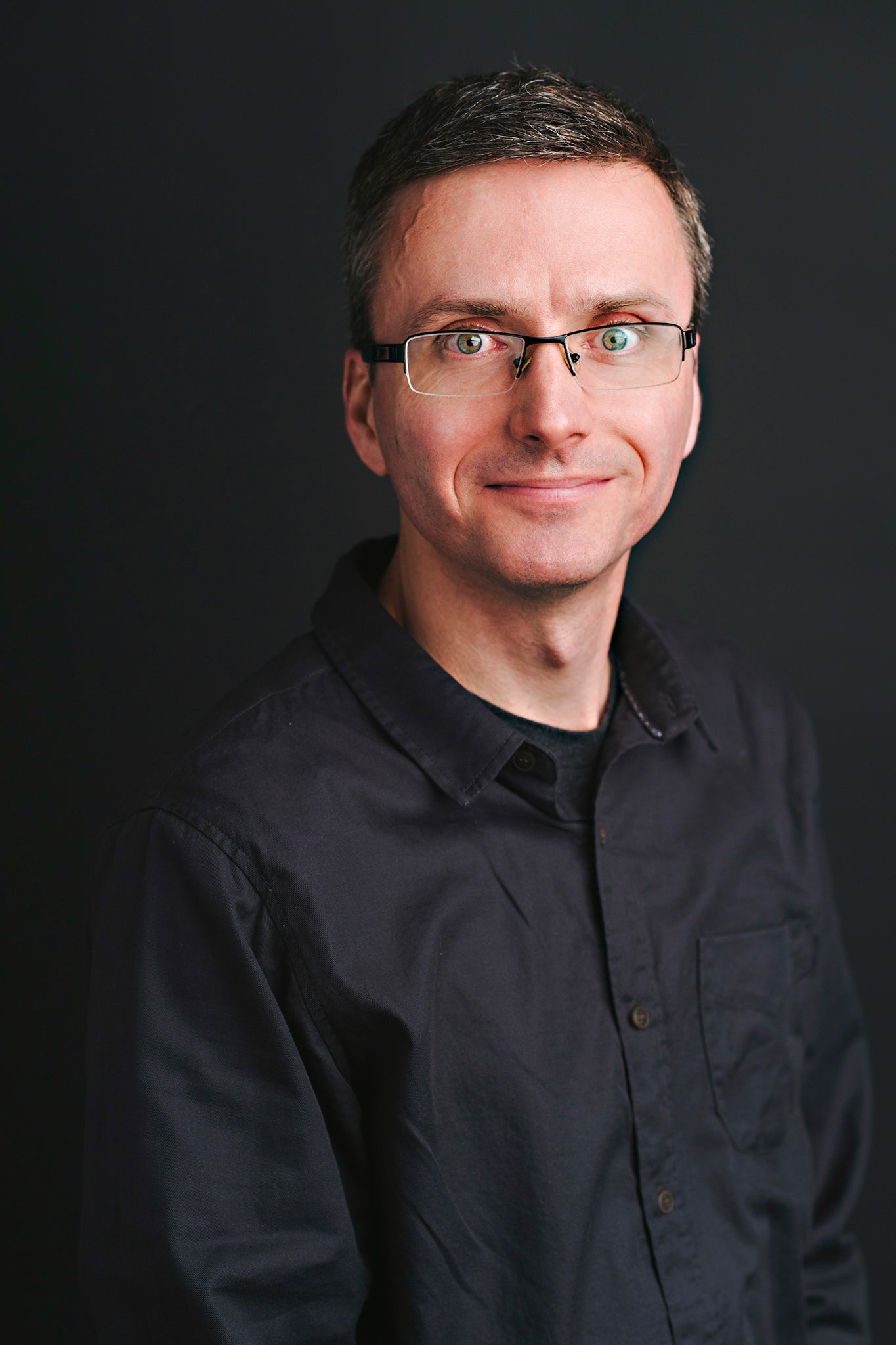
Climate change is making wild weather increasingly common—and raising the stakes for weather forecasters.
When we have accurate predictions about windstorms and heavy rains, we can prepare and protect our communities. Yet, precision in weather forecasting remains frustratingly elusive.
One of the main limitations, according to Professor Michael Waite, is the scale on which typical weather models run. These models represent wind, temperature, humidity and other quantities on a three-dimensional grid of points. The spacing between points in these grids is typically quite large, which means crucial weather effects are not represented.
“The typical grid spacing that Environment Canada would use is a few kilometres or more,” says Waite. “The problem is that lots of turbulent eddies in the atmosphere are smaller than a few kilometres, so none of those eddies are captured by their simulations.”
Waite compares weather grids to digital photos. “If your photo resolution is too low, then you can’t see the small stuff.”
Yet, reducing the space between grid points requires tremendous computing power and is ultimately too costly.
This is where Waite’s research comes in. Waite runs idealized experiments on supercomputers to better understand vital fluid dynamics, such as eddies, thunderstorms and gravity waves, on a small scale. He then looks for ways to improve the representation of these small-scale effects in weather models.
“If you take the digital photo analogy, we’re trying to do what they did on CSI where they take a low-resolution photo, zoom in, and estimate what’s happening below the pixel scale,” says Waite. “We look at a low-resolution simulation and say ‘Oh, at this point, we're going have a lot of mixing.’ We can't actually see the mixing, but we can infer it by what’s happening on the large scales.”
The impact of his innovative work could be far-reaching. “The end goal is always to have a better parameterization that might get taken up by people who actually do weather forecasting, so that they can improve the accuracy of the forecasts,” says Waite.
But his work could also enhance climate modelling, enabling climatologists to make more accurate and localized predictions.
“This work would help downscale climate predictions to regional scales,” says Waite. “For instance, what does a 2-degree increase in global average surface temperature mean for specific regions, like an area of Southwestern Ontario?”
***This article originally appeared in the Winter 2022 Math E-Ties alumni newsletter and was written by Robin Morden.***