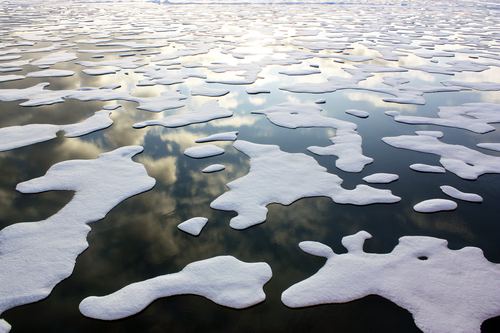
Chris Bauch, an applied mathematics professor and University Research Chair, recently published a paper in the Proceedings of the National Academy of Sciences (PNAS) on new research related to a deep learning algorithm capable of detecting and predicting tipping points.
Bauch was part of a team of researchers working on the project, which has far-reaching implications for assessing the risks associated with climate change tipping points.
The method used to train the AI, however, involved tipping points generally, and not only those associated with climate change. This approach takes into account tipping points that exist in human and non-human systems including the stock markets, algae blooms and even epileptic seizures. A tipping point, in this sense, refers to thresholds beyond which rapid or irreversible change happens in any system.
“We found that the new algorithm was able to not only predict the tipping points more accurately than existing approaches but also provide information about what type of state lies beyond the tipping point,” Bauch said.
The approach gains its strength from hybridizing AI and mathematical theories of tipping points, accomplishing more than either method could on its own. After training the AI on what they characterize as a “universe of possible tipping points” that included some 500,000 models, the researchers tested it on specific real-world tipping points in various systems, including historical climate core samples.
Deep learning is making huge strides in pattern recognition and classification, with the researchers having, for the first time, converted tipping-point detection into a pattern-recognition problem. This is done to try and detect the patterns that occur before a tipping point and get a machine-learning algorithm to say whether a tipping point is coming.
Now that their AI has learned how tipping points function, the team is working on the next stage, which is to give it the data for contemporary trends in climate change. The deep learning algorithm could act as an early warning system against runaway climate change.
Some tipping points that are often associated with run-away climate change include melting Arctic permafrost, which could release mass amounts of methane and spur further rapid heating; breakdown of oceanic current systems, which could lead to almost immediate changes in weather patterns; or ice sheet disintegration, which could lead to rapid sea-level change.
The paper “Deep learning for early warning signals of tipping points,” was published in the journalProceedings of the National Academy of Sciences (PNAS): https://www.pnas.org/content/118/39/e2106140118