Researchers have developed a method that can identify future COVID-19-like viruses within minutes, which could allow for quicker development and deployment of vaccines and treatments.
In a new study, researchers at the University of Waterloo used a machine learning-based alignment-free approach to accurately and rapidly identify and classify COVID-19 virus genome’s relationship with other viruses.
Unlike alignment-based approaches, the novel method requires no specialized biological knowledge of the organism being assessed or specific genetic annotation, and it can be used to both identify and classify any new organism, including synthetic ones with a high degree of accuracy.
“With this method, when there is a new virus like COVID-19, we will be able to identify what it is more quickly, enabling us to start working towards vaccines and treatments,” said Lila Kari, a professor at the Waterloo’s David R. Cheriton School of Computer Science. “Now that we have this technique, if another virus like COVID-19 was to affect the human population, we will be better prepared."
“In a few hours or even minutes, we will be able to figure out what the virus is related to and, therefore, how alarmed we should be.”
Researchers trained machine learning algorithms on the around 5,300 available viral genomes from the National Center for Biotechnology Information (NCBI) database, and used a decision-tree approach for successive rounds of training and refinements of classification.
Rather than aligning and comparing a particular COVID-19 viral gene with that same gene in other viruses, as done in classical alignment-based methods, an alignment-free approach was used. This novel method essentially extracts characteristics from the entire COVID-19 virus genome and compresses them into what the authors call a numerical “genomic signature”. The COVID-19 viral genomic signature is then compared with the genomic signatures of all known existing viruses, to determine their relatedness.
This alignment-free approach, combined with machine learning, classified the COVID-19 virus as belonging to the family Coronaviridae, genus Betacoronavirus, with 100% accuracy, and concluded that its genome is most closely related to three other bat virus genomes.
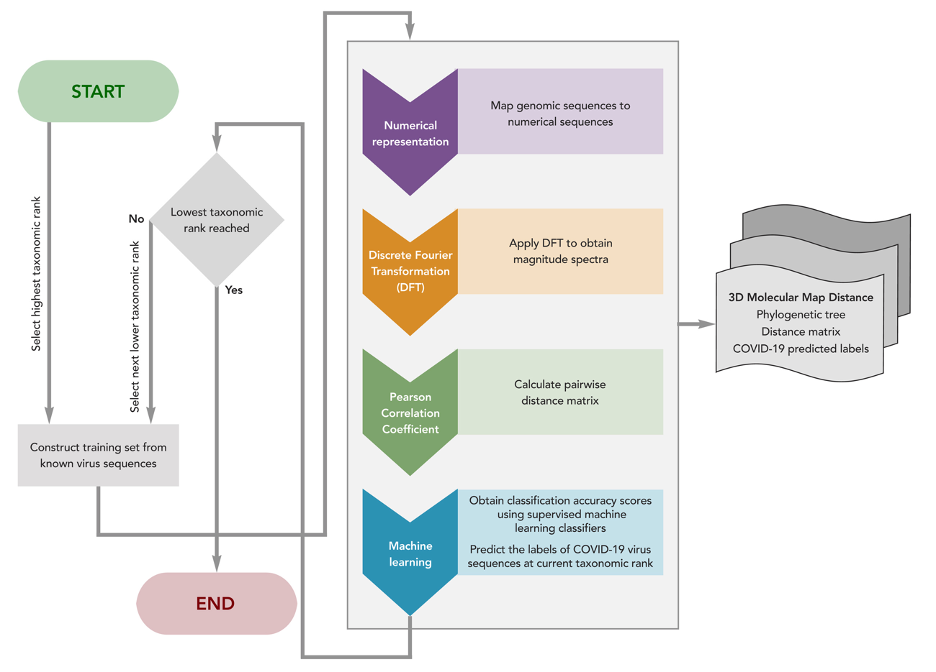
Machine learning combines with digital signal processing, resulting in successions of increasingly accurate classifications of the bare-bones COVID-19 viral genome.
“As recent events have shown, rapid identification of pathogens is of utmost importance,” said Kari. “While we cannot turn back the clock for COVID-19, hopefully having instant identification tools will help determine the potential seriousness of future outbreaks.”
A study detailing the new method, titled “Machine learning using intrinsic genomic signatures for rapid classification of novel pathogens: COVID-19 case study”, authored by Kari and University of Western researchers, Gurjit Randhawa, Maximillian Soltysiak, Hadi El Roz, Camila de Souza and Kathleen Hill, was recently published in the journal PLOS ONE.